Redefining OEE for the 21st Century
A version of this article was originally published in Reliable Plant.
Most plant professionals know that overall equipment effectiveness (OEE) is a summary metric of your equipment’s total productive output, or what you can actually use or sell. Manufacturing companies typically operate around 60 to 65 percent OEE. World-class OEE is 85 percent or better. While OEE is an excellent calculation to benchmark productivity, it needs to be redefined for the modern era.
The Origins of OEE
OEE’s origin was actually human before it was machine. While the term was coined in the early 1980s by Seiichi Nakajima, the founder of total productive maintenance (TPM), the concept was rooted in the work of Harrington Emerson, an American efficiency engineer whose research culminated in his 1912 book, The 12 Principles of Efficiency, which focused on optimizing efficiency as a whole in industrial work.
One of the main benefits of the OEE calculation is that it allows different types of businesses to be compared, identifying the magnitude of underlying problems.
The issue, though, is that the calculation is highly tied to the machines. This view of the world that stemmed from Nakajima’s work has resulted in most companies thinking about the people on a manufacturing floor as being a part of the line on which they work.
This made a lot of sense when humans were making far more diving catches to improve the output of unreliable machines. It was an optimistic view of an industrial world that almost assumed humans were performing perfectly and wrestling the best performance they could from their unruly equipment. Indeed, the six big losses that Nakajima catalogued were all arguably machine-based:
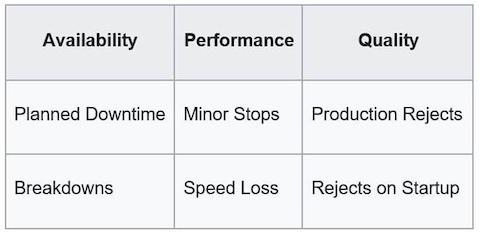
The Need for a More Modern Calculation
The problem with the current OEE formula is that machines are roughly 20 times more reliable than they were a few decades ago, according to a recent study by the Academies of Sciences, Engineering and Medicine.
If you dig deeper and look at just large industrial motors, only 3.4 percent fail on an annual basis – and half of these are in just 17 percent of plants. This suggests these “laggard” plants should be factored out. The bottom line is the machines can no longer be blamed.
More importantly, the picture of a factory today is much different than what was considered state-of-the-art in the 1980s.
Some of the key differences include the following:
More Products – Plants are being continuously reconfigured to manufacture more products, many of which the original equipment was never intended to make. This means humans now must be trained and remember lots of configurations and settings to manufacture all these products as planned.
Fewer Trained People – In many plants, the workforce is aging out; the percentage of workers in a labor union who are older than 45 years is 64 percent. Younger workers who are now entering manufacturing are learning complex systems from scratch and may not benefit from the years of apprenticeship and tacit knowledge their elders have.
More Reliable Machines – As mentioned previously, the modern factory is less about the diving catches humans do for machines, but rather the ones they do for other humans.
So, there are less experienced people doing more complex jobs with both more sophisticated and more reliable equipment. If you then break down OEE as it has traditionally been calculated, it is still accurate but less able today to point to the underlying problems.
The “Errors” in Today’s Plants
If you consider what seems to go wrong in a factory today, the sheer complexity of making lots of products and knowing what to do every time you start producing a new product has become too much to store in your head.
When you reconfigure machines to make new products at pace, crazy things can happen. Settings may be wrong, new supplies may be loaded incorrectly, jigs and parts may be inserted incorrectly.
Plant managers and supervisors often talk about hotspots on a production line. These occur when the equipment has built-in flaws or when reconfiguration has caused anomalies that result in machine breakdowns.
An example of this would be a cereal plant where a conveyor wheel would jam at a certain point on the line and need to be treated with mineral oil on a daily basis. This was not so much a machine flaw but rather an artifact of its use for another product.
It was a known and manageable preventive maintenance issue that the individual operator could address, but if it didn’t happen, the line broke down and potentially a whole town didn’t get their raisin bran cereal that week.
It might be easy to view these issues as human error, but it isn’t that simple. Quality guru W. Edwards Deming taught that 94 percent of errors come from the system itself, and only 6 percent are generated directly by humans. By “system,” he meant the set of instructions and work environment that the management team set up for the workers.
So, if it isn’t the machines and it isn’t the workers, then it is the management and industrial engineers who designed the contraption (the factory) and the theory of how to run it (the work instructions) in the first place.
OEE 2.0: Factoring in the Human Dimension
However, that’s also too easy of a pronouncement. The modern factory and its requirements to operate smoothly are complex. Add lots of products, and you have a mess in the making that is very difficult, if not impossible, to get right the first time.
What you must determine is how to embrace this reality and optimize it. You must augment humans so they can do more work accurately with less upfront knowledge and execute with precision.
Through data, opportunities for continuous improvement will be revealed. If you look at your business in this light, you can see the micro-losses that can be reduced or removed.
A revision of OEE – arguably, OEE 2.0 – should delve into the source of these micro-losses so teams can focus on eliminating root causes.
Ultimately, you should seek to eliminate what is preventable – errors caused by the process or workstation design, incorrect or missing procedures, missed training, supply-chain gaps, etc.
With emerging technologies that can produce granular data about the work performed by humans, these micro-losses can be exposed for the first time. You now have the power to recognize, categorize and prioritize them for investigation and elimination.
While proposing a new calculation for OEE may be premature, we certainly are ready to more finitely identify challenges and take action. Someday, you may once again be able to blame a humanoid robot for causing a problem, but in the early part of the 21st century, the machines are nearly blameless because they are pretty darn good.
It’s where we (humans) place them, how we configure them, how we operate them, and how we maintain them that needs to be assessed – this time with data.