Save Time and Make Money with Artificial Intelligence
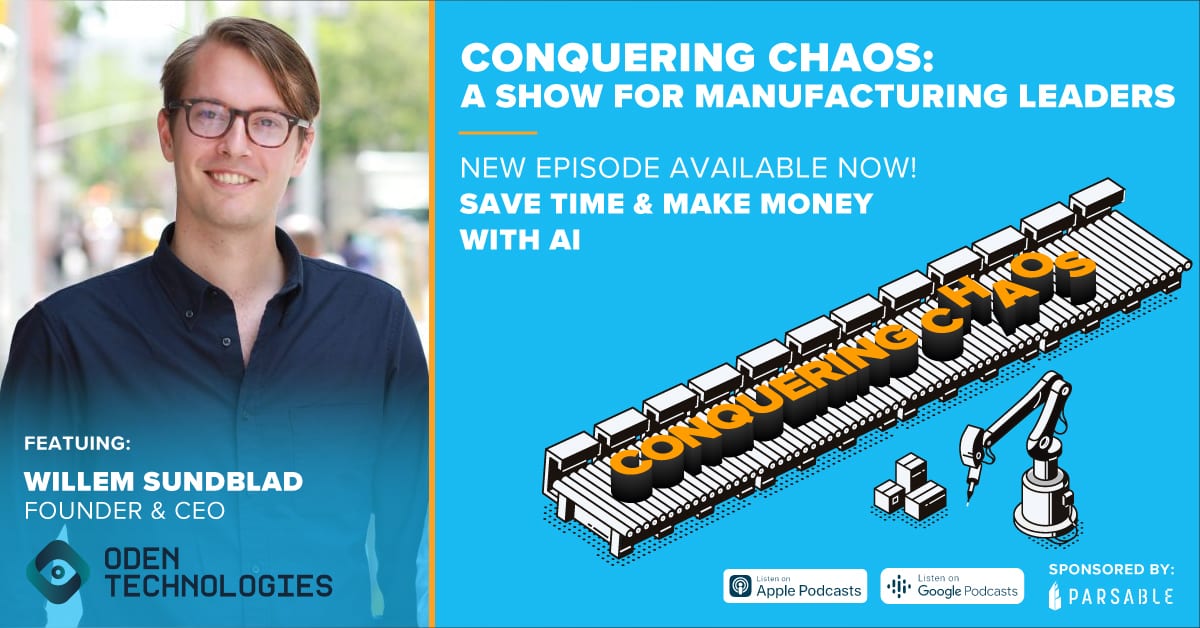
If your understanding of artificial intelligence (AI) comes from sci-fi movies like iRobot and… well, AI, then you should tune in to this episode.
Willem Sundbland, Founder and CEO of Oden Technologies and an expert in smart manufacturing, joins us to break down what AI actually is, what it isn’t, and what it can do to improve your organization.
We discuss:
-How AI and machine learning (ML) speed up tedious processes
-The difference between supervised and unsupervised ML
-What people get wrong about AI and ML
-What needs to be in place to implement AI and ML at scale
If you have any questions, email Willem at willem@oden.io and Josh at josh@parsable.com.
______________________________________________________________________________________________________________
Are you ready to start your digital transformation journey? Request a demo today.

______________________________________________________________________________________________________________
Check out the full episode below:
[00:00:00] Willem Sundblad: AI is never the silver bullet to the solution itself. It’s all about how you’re speeding up existing processes.
[music]
[00:00:07] Josh Santo: Welcome to Conquering Chaos, the show for manufacturing leaders. In each episode, we’re connecting you to the manufacturing leaders of today who are driving the innovations needed to future proof the operations of tomorrow. If you feel like your time is spent fighting fires, and try to control the everyday chaos, this show is the show for you. My name is Josh Santo, I’ll be your host.
All right, welcome to the show. Our next guest is impressive to say the least. He’s an expert in smart manufacturing, analyzing the latest trends in intelligent manufacturing. You can actually see some of his work published on Forbes.com. Aside from keeping manufacturers informed, he’s the CEO and co-founder of Oden Technologies, which is a manufacturing analytics company focused on driving quality and performance improvements to manufacturers through their AI powered platform. Everyone, please welcome to the show Willem Sundblad. Willem, thank you so much for being here today.
[00:01:08] Willem: Thank you, Josh. Thanks for having me.
[00:01:11] Josh: Really excited about that this one and we’ll introduce the topic in just a second. I like to start the show, really, by making sure we properly introduce the guest. It’s not enough to just have a background like we went through, but as the CEO and co-founder of a tech company, a tech company focused on the manufacturing sector, I should say, tell us about what a day in your life looks like in this role.
[00:01:36] Willem: Well, right now, I’m still working from home. I’ve got a wife and a one year old child here. If you hear any baby noises in the background, I apologize, but that is very much part of day in the life of me now. I’m currently living in Vermont, so when I’m not working, I try to ski as much as I can. When it comes to Oden, I spend probably about a third of my time focused on product reengineering, a third of my time just as a people leader, and a third of my time talking to customers, or partners trying to help them along their journey on smart manufacturing and see where we can help.
[00:02:16] Josh: On that topic of talking with your customers, what kind of conversations are you having?
[00:02:22] Willem: A very common topic is– These are big organizations with hundreds of plants, they may have realized, especially in the last year that they need to transform, but it’s a really big task. I’ll just give you an example of a customer that I talked to that has hundreds of plants. If you install one factory per month, you’ll be in a deployment for over 20 years. How do you actually transform an organization like that? How can you create the right type of program that both gives them the long term vision that they want, while decreasing risk and eating the elephant a couple of bites at a time, to really show both ROI, show the people change management, and show them, here’s what’s possible today and here’s where we’re going in the future.
Diving in a little bit too much into the topic already, but one of the key things to get across here is that AI is never the silver bullet or the solution itself, it’s all about how you’re speeding up existing processes. A lot of times a customer might have a high level idea of what analytics and AI can do, but it’s really about tying into, what’s the business goal that they want to achieve? In most cases it’s, “I want to increase my output with 10%, but I don’t have the money to buy 10% more production lines, or buy 10 more factories.” How do you tie the technology to the real business outcome that they will drive?
[00:04:04] Josh: That gets into a little bit of the transformation, how to use technology at scale, within a reasonable timeframe, that actually makes an impact, that still gets you towards your vision. There’s a lot to be factored into that, and you mentioned AI. Today’s topic and conversation, we’re talking about artificial intelligence and machine learning. Now, I want to get into this, and I have to be honest, I’m really going to play the part of someone who’s uneducated on this subject. I promise, I’m just playing a part right now.
Trust me when I say that I totally know everything you’re talking about right now.
No, all jokes aside, when I hear artificial intelligence and machine learning, my mind goes to science fiction. They have me as someone who’s even worked in this industry. I immediately think of, in particular, the movie I, Robot that that came out I think in the early 2000s, starring Will Smith. I know that that’s not what we’re here talking about today, but can you just level-set and help us really understand what artificial intelligence is, what machine learning is?
[00:05:14] Willem: Yes, happy to. I’ll start off by saying that the science fiction idea of artificial intelligence really focus on general artificial intelligence, where you truly have a sentient being who has their own thoughts and ideas, and takes actions and plus those actions. What we’re really talking about here is narrow AI, where it’s focused on a specific use case.
The difference between AI, machine learning, and also deep learning, you can almost think of it as Russian nesting dolls, where all deep learning is machine learning, all machine learning is AI, but not all AI is machine learning. One of the ways that we can make machine learning a little bit more real for people is, let’s say that you’re running a manufacturing organization. You know that if a certain metric goes above a certain threshold, that creates bad quality. That’s a known problem and it’s very easy to prevent, because you can just put in the normal rules based alert, and you can prevent that.
Where machine learning is really valuable is where the rules are either unknown or they are difficult to figure out. What the machine learning system can do is really look at the pattern of data and understand here’s something that’s different. You usually talk about either supervised machine learning, or unsupervised machine learning.
Supervised machine learning, is when you’re showing the system, a bunch of different examples to say, “Here’s what I want to find, I want you to look for similar examples like this.” That’s a typical example of what we can do in quality analysis. We’re showing the system many times where a quality issue has happened. We can then learn the rules for what creates those types of quality issues. We can then alert and prevent them. Just learning the rules is really valuable for the customer. Obviously, alerting and preventing them makes it even more valuable, because then you can just save that lost production.
Unsupervised learning is when you don’t really know the rules at all, but what the system can do is cluster things that are interesting. It might be that you can say, your production from this supplier always has a different quality output than this supplier or this shift is showing up like this in your performance report compared to this shift.
It’s more about finding the similarities or finding the patterns in the data that might be really complicated for people to do. A lot of this actually, when it comes to complicated, a lot of this is things that would be theoretically possible for a person to do given all the time and money in the world, but people don’t have that. That’s where the computation powers are really helpful because you can help people find the needle in the haystack, you can help them find where to look for problems, and really be a partner in driving business outcome. It’s never about people or AI, it’s really about the combination of the two, that makes the whole system more powerful.
[00:08:38] Josh: A lot of interesting points in what you just called out. I’m going to do my best to recap in the way that I understood it. Starting with the point that you made last, which is AI, machine learning, this is not a replacement for people, this is a tool to help people. It should speed things up, it should take out some of the tedious, while maybe interesting research that has to happen to understand all these different variables that come into play, that actually go into a certain event happening.
That’s such a key thing that I think needs to be understood, because when we talk about like the future of manufacturing, there is still concerns about what does that mean for people? Are people going to be replaced? When you start talking about artificial intelligence in particular, and all the connotations from how it’s been popularized in science fiction, people start to think, “Well, where do the people fit in?”
I love that one of the main messages you’re making here is that artificial intelligence, machine learning, these are tools that can help workers do the job that they are expected to do, even speed up, especially when it comes into that point that you made about like, “Look, people can technically gather all these data points in review all these data points and understand what are the variables and what are the constants with all of these data points. To do that takes a significant amount of time, that amount of time then it impacts what you can do with that information.” I love that concept.
Now, talking about machine learning, the supervised and the unsupervised, if I understood correctly, supervised machine learning is, we know these series of events, like these things that happen, lead to this outcome. Then that’s essentially the rules, meaning if this then this. From there, learning the rules, going through the cycles, understanding the data points that formulate those rules, the machine learning can start to flag, something’s different here, something’s different here, which allows you to respond more quickly.
The unsupervised, it sounded like, that’s just taking in all the data points and pattern matching saying, “When these variables are true, this tends to happen,” or whatever the case may be.
Is that an accurate summary of some of the concepts or is that missing the mark?
[00:11:09] Willem: Yes. To simplify, I would say, in supervised learning, you have a goal in mind that you’re saying, “Here’s the example that I want you the system to learn. Then show me other times that this happens, or show me this time as it’s happening.” In unsupervised learning, you don’t necessarily have a goal in mind but you’re telling the system to show me patterns. The system then clusters, this thing looks like that, like, out of all your runs, here are the commonalities between these things. They’re both really valuable, but they might serve different use cases.
[00:11:47] Josh: Is the goal with unsupervised machine learning to eventually get it to a point where it is the supervised learning or it does become that known and no longer is it an unknown, now, it’s an understood rule or process.
[00:12:05] Willem: They can certainly serve different purposes. It might be that an unsupervised model can help you uncover the next place to look in order to find those rules, that’s certainly a possibility. Because, a lot of time, we call that guided discovery, because when you have quite few engineers in the factory, but a tremendous amount of data, it’s really hard to know where to look and they might be looking at just the immediate fire instead of the long-term strategic problem. Unsupervised techniques in clustering and planning patterns, can again, just speed up the problem solving or speed up the discovery for the people who are going to take the ball and run with it.
[00:12:57] Josh: Okay, great. I appreciate that clarification. Now, one thing that’s still not completely clear to me yet– Machine learning, I think I got it at least as far as a basic understanding. Now, how does artificial intelligence come into play?
[00:13:10] Willem: That’s where all machine learning is artificial intelligence. You use the terms differently, depending on who the audience is really. All machine learning is artificial intelligence, all artificial intelligence is not necessarily machine learning. AI is broader. You might go into other techniques, such as planning and reasoning, that aren’t necessarily supervised or unsupervised machine learning techniques.
[00:13:44] Josh: Understood. Machine learning is a key part of artificial intelligence, but it’s not the whole thing. It doesn’t represent the whole. Understood. These are two distinct concepts to agree. They’re definitely related, but they do have their own definitions, what you called out being the hierarchy of it, because one of the things I was wondering was, if it was like the classic song, Love And Marriage, where you couldn’t have one without the other. It sounds like you can’t have machine learning without artificial intelligence, because it’s a piece of it, but can you have artificial intelligence without machine learning?
[00:14:28] Willem: Yes, you can. There are artificial intelligence. AI has been around for a long time. Even older techniques like rules engines, expert systems, those things are considered AI but they’re not using machine learning.
[00:14:54] Josh: Okay, well, that’s actually a good point. Stepping away from some of these more technical definitions, how would someone who is by no means an expert, like myself, with machine learning and artificial intelligence, what are some examples in our everyday lives as consumers that you would point to that say, that’s a good example of these topics?
[00:15:18] Willem: If you have a smartphone today, you’re constantly interacting with AI actually. If you go into your Photos app on your iPhone, it can already segment different people who are in different photos automatically. That’s through machine learning. Sometimes if you just pull down a little bit, the iPhone now makes recommendations on things that you probably should do right now. Like, if I pull down, it says, send a message to my wife, maybe it’s because I usually do that at this time, that’s a rule that the phone actually learned.
Another example, on the phone again, it’s just the facial recognition. Images, especially, is a field where AI has become really good. Outside of your normal day-to-day, if you’re looking at MRI scans, as an example, machine learning systems have been proven to be more effective than doctors at diagnosing tumors. The most effective is actually when you have doctors and AI systems collaborating. It’s already happening in many different fields. It’s, again, this idea of narrow AI, where it’s a specific use case, where the system speeds up an existing process.
[00:16:45] Josh: Understood, those are such great examples, because as soon as you called it out, I literally felt in my head a click. That click of getting it, you are right, because you started describing the photos, as soon as you mentioned you could type in someone’s name, and it’ll pull the picture of them, I was like, “Machine learning, machine learning.” Great.
Well, you called out, also a key thing, which is like this is prevalent around us, a lot of this stuff isn’t really that new. The topic itself isn’t new, it’s definitely been touted in thought leadership circles. What I want to do right now is I want to talk about the perception of artificial intelligence and machine learning, as well as some misconceptions surrounding it. Let’s start first, Willem, what do people get wrong about AI and ML?
[00:17:40] Willem: So, I actually see people either think it’s magic or bullshit. It’s neither, and it’s really important. I think that goes back down to the concept of narrow AI, and really understanding what is the problem that you’re solving and do you have a way to effectively model that problem? Then can you do that in a way that’s efficient to scale? Again, it comes back to the business process again, because if you’re looking at manufacturers, they have tons of business processes, you’re not trying to invent something new, you’re not trying to create a new process. Because let’s say that we’re trying to optimize quality, we’re trying to optimize performance, there are people that are doing that, every day. The key is to identify where are the parts of that process that you can either automate or augment with technologies like this.
Going back to your everyday example, again, if you want to find photos of a relative on your phone, you could do that yourself if you look through every single one of those photos. It’ll take you a long time, it will probably take you so much time that you won’t even do it. But, with the system, you can now just go to your phone, and you can say, “Grandma,” and then you get all the photos of grandma. Now, you’re going to do that because it’s just so easy. There’s parts of this where by automating and augmenting the process so that it’s easier, you’re allowing people to do something that they didn’t do before because it was just so prohibitively time consuming before.
[00:19:18] Josh: Yes, essentially, you’re giving people time back, which, time is a huge constraint on capacity. Every manufacturing facility I’ve ever gone to, I’ve yet to meet the person who has nothing to do or who has the bandwidth to take something else on. It’s a big thing, especially when we start thinking about some of the other industry trends of look, there are shifting workforce dynamics where there’s a major concern, “Can we even bring people in?” If you can’t bring people in, you’re going to be tasking the people who are there to do more. Really, you need to explore those options that are going to help you do more with less and just be more efficient with the time that people spend out there in the facility.
[music]
Hey, we’re going to take a real quick break to hear from our sponsors. Stay tuned for more Conquering Chaos.
[music]
Hey, this is Josh from Conquering Chaos. Here’s another free tidbits sponsored by Parsable that can help you make quick and sustainable changes to frontline operations, get rid of paper. You probably don’t realize how much inefficiency and waste is caused by using paper based forms, checklist, and SOP because it’s been normalized over time. While with modern day technology like smartphones and tablets, you can bring a dynamic digital experience to operators and mechanics while unlocking opportunities to drive sustainable improvements across your facilities worldwide.
Think about it this way, you use your iPhone for everything in your life; communicating with people, looking up information you don’t know, and interacting with pretty complex systems or Wi-Fi-enabled household devices. You live connected, we all do, and we rely on it, but most frontline operations lack the tools and technology to empower people to work like they live.
Connected. Parsable’s Connected Worker platform brings your team the tools needed to make work digital and make frontline workers connected workers. Connected workers are connected to people, information, systems, and machines they need to work with to get the job done right every time.
Go digital, get connected, and transform your operations at scale with Parsable by empowering your frontline workers to work like they live. Connected, try 30 days for free today.
[music]
Now, back to the show.
Now, where do you think, like some of these misconceptions come from? This idea that it’s either magic or bullshit, why isn’t there a more practical understanding?
[00:22:01] Willem: I think it’s just that we have– The idea of AI, or a lot of the techniques have been around since the 50s and 60s. Then there was something called the AI Winter, when people discovered all these tools and technologies, but the computational power and supporting technologies just wasn’t there. Now, you have a confluence of events where we know it works and it’s possible to do, but still the hype led the transformation; like people expected it to be bigger and faster.
I think especially also, for a long time, it has been consulting based science projects rather than maybe scalable solutions, and that’s a big difference there. I think maybe a little bit what you started with saying that it’s I, Robot when it really isn’t and we then say, “No, it’s not I, Robot, but I can help you predict this quality failure.” It’s almost like, “Oh, well, that’s not what I expected it to be,” but actually, it’s more valuable to have a good quality of prediction on your manufacturing line than to have I, Robot on your facility.
[00:23:19] Josh: That’s an interesting point. It sounds like AI went through a bit of the dark ages before meeting a renaissance, and that was just due to what was available to support it. I like this idea that you’re talking about of a scalable, realistic, practical solution, not a science project. Now, you’ve mentioned before that it should be a business process that’s already well-defined and it’s well understood. I guess what I would like to do is make sure that people understand maybe where the gap is between practical implementation versus this science project that you brought up, which I’m sure a lot of people have discussed in one way or another, or try to sell others on in one way or another.
[00:24:11] Willem: I think a key thing there is really about time to value. I’ll take a practical use case. We do this thing called Golden Run where we’ll look at the way of manufacturers processing a given product or a family of products, we can find what the efficiency potential is, and prescribe them the right set points to get there. On a product by product basis, you can get 5% more output from this product. If you change your temperature to be this, your motor RPM to be that and your speed to that, etc.
Now, if you take the science-based approach, it might be a couple of months before you have the answer, because you’re doing all the data collection, then you’re doing the modeling, and then you might get a result, but you don’t really have a way of taking action on it in real time.
There’s a shelf life there as well, because if you took data from a few months back, it may not be relevant anymore because things may have changed. The environmental conditions in the factory may have changed, the suppliers may have changed, the tolerances that the customers need may have changed.
You have to have a reinforcement learning and a real-time process around this of, how do you constantly add in new information and how do you make sure that the people are taking action on it as fast as possible? Value only comes when the person takes a different action than what they were doing before, because if no one is changing their actions, there’s no value. So, you need to change people’s actions.
That again goes back to the business process of how do they make changes today and where in that process can you speed it up by showing them new information, better information and automating loops in between those people as well, so that you’re helping with their communication.
[00:26:04] Josh: Understood. The more practical and specific that you can make it, here’s the defined problem, or even not even necessarily a defined problem, because really you’re just examining the process and you’re wanting to collect the knowns and the unknowns.
Now, the big point that you’re making is that data also has a shelf life. It technically expires or at least the value that you can get from it can expire under certain conditions. Meaning, look, if you don’t take action, a variable changed in between when you first collected it, that data point and now, that impacts the results one way or another. If you don’t have that ability to quickly get the information, understand and interpret what that information is indicating, and then do something with that, you’re not going to get any value out of something like this, out of a tool like this.
[00:27:01] Willem: Yes. So much really just comes back down to the time to value. A quote that I really like is from one of the co-founders of Spotify, where it says that the value of a company is the sum of all the problems that you’re solving. I think that’s extremely true especially for manufacturing companies. It’s like, if you have a faster rate of solving problems than your competitors, you’re going to be able to solve more problems, you’re going to be more efficient, and you’re going to be more differentiated.
A lot of this goes down to the rate of learning that an organization has or rate of problem solving, which again is really linked to the skills gap and some of the people concerns that folks in manufacturing have, because if you’re currently understaffed and you have been for the last three years, it is not realistic that you’re all of a sudden not going to be understaffed. It’s better to figure out how you can make sure that the people that you have can be as effective as they can be, and really spending their time doing the most high value tasks. That’s just a classic lean manufacturing principle. It’s just like cut out non-value added tasks. A lot of these things are, how can we cut out non-value added tasks or the low value added tasks so that the people can spend the most of their time doing high value tasks?
I’ll give you an example again, from manufacturing, which is very common. It’s very difficult because a lot of systems are asking a lot from operators. Operators already have a demanding job that isn’t super well paid or has a lot of demands. Just as an example of clocking, all types of downtimes and all reasons for downtimes, it’s something that’s really important for the organization to learn, but it’s a new nuisance for that operator because they’re either doing it in a different system or they’re doing it in pen and paper, but that is a typical example where machine learning can be really useful.
Just looking at the pattern of the data to actually detect if it’s a downtime and for what reason it is a downtime, because then the operator doesn’t have to care about the reporting of what’s happening. They can just focus on figuring out how they prevent it, or how do they get it back up and running again. You’re increasing the validity and the integrity of the data for the analytics and for the executives of what’s going on on the factory floor while making it easier for the operators to just do the jobs that they want to do.
[00:29:58] Josh: There were two. very critical topics that you just brought up. One was, how AI and ML can contribute to your competitive advantage, and two, the other one is focusing on this gap that a lot of manufacturing companies are experiencing between experienced and inexperienced, just staffing in general. That idea, you put it very specifically like, ”Look, it’s not going to all of a sudden go away, so you also need to focus on how do you do more with what you do have. You do that by eliminating that low to no value-added tasks, those nuisances that are very necessary from an operational perspective, like the example you gave of reporting unplanned downtime, but ultimately a pain. By freeing up their time, making their experience just a little bit more enjoyable, what kind of impacts those could actually have on an operation, not just from the morale side of things.
Especially when we talk about how it’s a very competitive industry from getting talent perspective, you are absolutely seeing workers coming into that ability to be a little bit more picky and choosey about where they spend 8 to 10 to 12 hours of their day, 4,5,6,7 days a week. Being able to say that you’re going to be able to do what you like to do, and a lot of these pain points that you have of having to do these things that we require, just because we don’t really have a good way of doing it another way, so it all falls on you to work around our current gaps, that’s gone.
Those are two interesting, I guess, I would say value propositions of a tool like this, where you may not be considering it, but think about how this change in the way of doing work leads to something that you might not be able to quantify as hard as let’s say producing more units, for example.
[00:32:11] Willem: Yes, and especially one leads to the other, because if we are talking about the business process and the value and the business outcome that we want to drive, talk about increasing OE or increasing efficiency for the company, you want to drive output improvement, you want to drive more first pass yield, more utilization. That’s what you want to drive, but it’s a lagging in indicator because you first want to make sure that the people can be more effective with their time, which drives the output improvements, so the first pass yield improvements and everything else.
[00:32:48] Josh: Absolutely. Well, look, I know that I’m pretty much sold on the idea of artificial intelligence and machine learning. I was sold back when I first saw I, Robot, but clearly, I had a misconception, but I do want to talk about is this something– If you are interested in implementing artificial intelligence and machine learning in a factory or at scale, talk to us about what needs to be in place before you really move forward with something like this?
[00:33:17] Willem: I almost look at it as the Maslow’s pyramid of needs in terms of the infrastructure that you need. If you are completely paper-based in your processes, you’re not collecting data today, and everything is very much analog, you have a little bit of a hill to climb when it comes to the operational infrastructure, and there’s no beating around the bush around that. But, if you are on the paper-based route or if you don’t have your equipment networked, you probably have a lot of opportunity to improve your process, and that’s ultimately where the ROI comes from.
The way we look at it is, you need data in order to actually train these models, and make these recommendations, and to get the improved output. That usually starts with networking the process equipment so that you’re collecting the data, and it needs to go somewhere, whether that’s on a historian on the factory floor, or whether that goes to Kepware, and then on to a cloud database, but the data needs to be collected from the factory floor, one way or another. Usually, networking PLCs and pulling it into Kepware is usually the lowest cost and the fastest way of doing it outside of big historians.
We usually see that you probably want something like an MES. You’re going to need an ERP system so you know what’s being made at any given time, and/or an MES system so that you have the tracking of what’s going on as well. There is a base level of infrastructure that you need before you can start talking about advanced data analytics and machine learning use cases.
One thing that I think is important also is that it’s not 100% sequential. You want to have the analytics in mind as you’re building out [unintelligible 00:35:18] infrastructure because really, the high-value add is going to come from the analytics, not from the infrastructure itself. Because as soon as you’ve connected all those machines, you’ll be flooded with more data than the people know what to deal with, and so you need an intelligent way of actually taking action on it.
A normal, let’s just say a plastic extrusion production line, will throw off millions of data points per day. That is too much for an individual to analyze on their own to say, ”Here’s good, here’s a bad, here’s what we should be doing with our process.” That’s just one line. If you’ve got a whole factory, if you’ve got many factories, it’s just too much data for people to do proactive, strategically important analysis. It’s really good for firefighting because if you have a problem, the data is collected, you can solve it quickly because you know where to look.
Again, that’s where machine learning and advanced analytics helps drive an improvement in that process or speeding up the problem solving, because you don’t just want to solve the reactive problems and the firefighting and speeding up the firefighting, you want to prevent the firefighting in the first place. That requires more advanced capabilities in how you really drive, how should we be making our products in the first place?
[00:36:48] Josh: So, definitely need to take inventory of what those key systems and ways of capturing information are. There is a certain foundation that has to be had. What about–
[00:37:02] Willem: I’ll give you an example. For some of our products, we have just requirements. If you want Golden Run, our prescriptive analytics on performance improvement, the data that we need to provide that is we need to know what product is being made, we need to know the targets for that product, we need to know the process parameters that impact it, and then if it was good or bad. That usually means we’re tying into an OPC server to get that process data, we’re ingesting data from the ERP system on the targets for how that product should be run, and from the quality system to know if it was good or bad. Then we can really connect those systems, make that data useful, and provide the recommendations on top of it.
[00:37:47] Josh: Great, understood. One of the things we’re getting into, and it’s something I want to delve in a bit more. We spend a lot of time talking about artificial intelligence and machine learning, but we haven’t spent a whole lot of time talking about Oden Technologies. I’ll let you take it from here. You talked a little bit about Golden Run and what’s needed to get that setup, but talk to us just more in general about Oden Technologies, how artificial intelligence and machine learning comes into play here, and everything, or a little bit about everything that [chuckles] Oden Technologies can do to provide an impact for the customers you serve.
[00:38:21] Willem: Yes, happy to. We start off by ingesting all that data, or actually, even before then, we start off by making sure that we have a very clear business problem that we can solve, because it starts with does the customer want to improve their quality? Do they want to improve their first pass yield? Because unless you have a clear reason to do it, there’s no point really. We’ve found that we have the best success where there is real alignment that the customer wants to drive $100 million in efficiencies through quality improvements. Perfect, we know we’ve got a goal to catch.
[00:39:01] Josh: Do you find that customers already have that identified, or is it less defined and it takes a couple of conversations with you and your team to find what that goal is that should be focused on?
[00:39:14] Willem: Usually, they should certainly have what the high-level goal is. They should know that we as a company want to grow, or we as a company are struggling with quality, we need to improve our margins. At the highest level in the business objectives, they really should have that. If they don’t want to improve their business, they are probably not looking for Oden Technologies to help them anyways. [chuckles] I don’t know why we’re talking to them unless they want to improve their business.
The highest level objectives should already be in place. The key thing is then to tie, okay, well, how is what we’re doing on the factory floor in driving quality output, how is that going to translate to the bottom line? That’s usually more where the discussion happens.
Especially if it’s a new customer or even a new industry, what are the biggest quality problems? Because, there are industries where we have a lot of domain knowledge, so we might know that, but there are others where we just don’t. Then there is, you still have, even though a customer may have the necessary technology requirements, it’s usually still siloed. A huge value add in the beginning is just aggregating the data from those different systems, and making it easier to access for people while we’re also building in the more advanced capabilities.
Something like Golden Run, to provide those recommendations to improve output takes between one to three months of existing data to really build an accurate model. That can be you know, from the last three months, or if they haven’t collected before, if they’re just starting, it’ll take one to three months before we’re providing those recommendations. If the data is available, we can turn it on and deliver it within one to three weeks, from kickoff to go live.
It’s really about first identifying, what’s the real reason the customer wants to transform, just so that we know that we’re delivering value in a way that makes them recurring customer and a growing customer. It’s almost always focused on performance improvement or quality improvement.
Quality, again, the use case, there’s really interesting because there’s so many things that you can’t test when you’re making it, in manufacturing, you always have to take it to a lab test it and there’s a lag time and maybe God forbid, it goes to the customer anyways. That’s where we can really create those rules of understanding, you’ve got the real-time process data, you’ve got the offline quality data that comes in many hours later. If you marry those two, you can do a real assignment of where that quality problem happened, why it happened, create the real-time proxy metrics that can help the customer prevented in the future.
We were always adding new machine learning applications that drive new recommendations for customers, but today, the things that we’re delivering outside of just the normal data aggregation platform is prescriptive analytics for performance, predictive quality, or just OEE tools that tells you, “Here’s where you have the biggest opportunity to improve your OEE.”
[00:42:39] Josh: When you’re having these conversations, when you’re talking about data from other systems, are you running to any hesitancy from others to either connect to your platform or to share data from their systems to your platform?
[00:42:57] Willem: I’ve heard of that a lot more in other industries. In the industries that we’re in, the customer owns the machines, the customer owns their SAP instance and there are ways of really extracting that information. The connected enterprise means that you’re tying all these business systems together in order to get a more complete picture, so the integration questions becoming less and less of a question concern or technical challenge.
There certainly are industries that I’ve seen outside of our direct customers, where the machine landscape is very consolidated, where the machine maker might just own that machine and they’re not letting anyone get access to it.
I’ve seen that in the semiconductor industry as an example, where it’s just like, the machine maker just owns it, and they won’t let go that data because it’s their IP. That’s really difficult and I think that is a no problem. I doubt that it will be a problem in the future because I think that’s just a business strategy that is not aligned with what the customer wants to do so you can’t keep doing that for a long time. The data integration piece is getting easier and easier all the time.
[00:44:19] Josh: It sounds like there’s not as much resistance as one may expect to this idea of well, just integrating with a system like Oden Technologies and it also sounds like, correct me if I’m wrong, because integration is a critical part of the service that you provide, this is something that your platform does well.
[00:44:42] Willem: Yes, we do well when it comes to the data integration and aggregation, but we also rely on partners to do that. If a customer, if they don’t have the machine’s networked to a central location, we’re going to have a partner who’s going to help them do that piece of work, because we don’t do that piece of work. But marrying the data between that system, and the quality system, and the ERP system, that’s really where we come in.
[00:45:11] Josh: Understood.
[00:45:13] Willem: I think one- but to go into some of the challenges that I know customers see, because I don’t want to paint a picture that is too optimistic or too naïve here is that I think the thing that’s holding people back is that the integration and the OT infrastructure upgrades that they need to do, they do cost money and the cost time. If there’s an uncertainty in the ROI, that makes it a much more risky proposition.
That’s why it’s, again, so important to really focus on where’s the ROI going to come from? How big is it going to be, so that the customer can make a really informed payback decision, because this payback is faster than most of the things that they bought, especially when it comes to machines, or upgrades, or capex investments that they’re doing, you should be looking at within a quarter or at least the next two-quarter payback, otherwise, you shouldn’t do it. But then, that type of ROI is what helps justify the integrations.
[00:46:17] Josh: Well, I think one of the things that stands out to me from what you said, and you said this a little bit earlier, is that if you are able to provide one to three months of the data that’s needed, you’re looking at a one to three week potential turnaround time, which is incredibly fast for implementing not just software, but like new technology, especially, one of the points you brought up way earlier in the conversation, which is the challenge of how do you scale? How do you move fast and get this to everyone and start to get that value quickly? It sounds like that’s something that your team is well versed in handling and working through.
[00:46:57] Willem: Well, let’s just take a hypothetical manufacturer who has many, many plants, and you can probably bucket those plants into, three degrees of connectivity. If you have a high degree of connectivity it’ going to be easy to implement, and it might be a one to three week lead time to do that. If you’re medium, it’ll probably take one to three months. If you’re high, it’ll take three to six months.
If you just look at implementation time itself, then you know that you have the fastest pay back on the high connectivity plants. If you roll those out quickly, the ROI from those plants can fund the infrastructure upgrades on the medium plants, then you roll those out, and the ROI from those plants funds the upgrades on the other ones, because there’s probably also a correlation between how much they’ve invested in their existing infrastructure, and how valuable those plants are for the business anyways. You’re starting with higher value plants, and then you go down the list. The project can fund itself pretty quickly.
[00:48:04] Josh: Understood. Looking at it high, medium, and low, and building that momentum of wins.
[00:48:11] Willem: Yes.
[00:48:12] Josh: Grouping that together, these are the wins great, now that we’ve built some success here, let’s take that to the next level, and then let’s take that to the next level. I love that
[00:48:21] Willem: The wins and the internal capabilities. Like who internally are the people who are going to drive the project? What’s the engagement between the vendor and the internal team? How do you drive that alignment?
[00:48:34] Josh: People are always critical, every conversation I’ve had with everyone about what change are you making in your company or what change are you helping bring? Inevitably the conversation comes down to you have to have the right people involved no matter what the tech is, no matter what the problem is, you got to find the right people. Almost the same as finding the right plant like you were describing too.
I think this has been a great conversation. Before we completely wrap up, if someone was interested in learning more about Oden Technologies, in particular, some of the things that you called out like the Golden Run, how would they find out more information?
[00:49:15] Willem: They can certainly find more information on oden.io, our website, but they’re also welcome to reach out to me directly. My email is willem@oden.io. I’d be happy to talk more.
[00:49:28] Josh: Well, great. For those listening, really think about a defined business process that you would want to explore how machine learning and artificial intelligence can help you understand how to optimize that particular process, and how does that tie back to your overall business objectives? I think this has been a great conversation, Willem, anything that you wish I asked you that I did not ask you?
[00:49:51] Willem: No, I think it was great. Thank you, Josh. You did a good job.
[00:49:55] Josh: [laughs] Great. Thank you. I appreciate that. All right. Well, we are going to wrap. Please, if you’re interested reach out to willem@odentechnologies.com. Of course, always feel free to reach out to me josh@parsable.com. Until next time, thank you for listening.
[music]
That’s the show. Thank you so much for joining us today. Conquering Chaos is brought to you by Parsable. If you’re a fan of these conversations, subscribe to the show and leave us a rating on Apple podcasts, just tap the number of stars you think this show deserves. As always, feel free to share what’s top of mind for you and who you think we should talk to you next. Until then, talk soon. Take care, stay safe, and bye-bye.
Listen to find out how you can save time and make money with artificial Intelligence