Lessons from AAM: Managing Data, Connecting Workers, & Breaking Down Silos
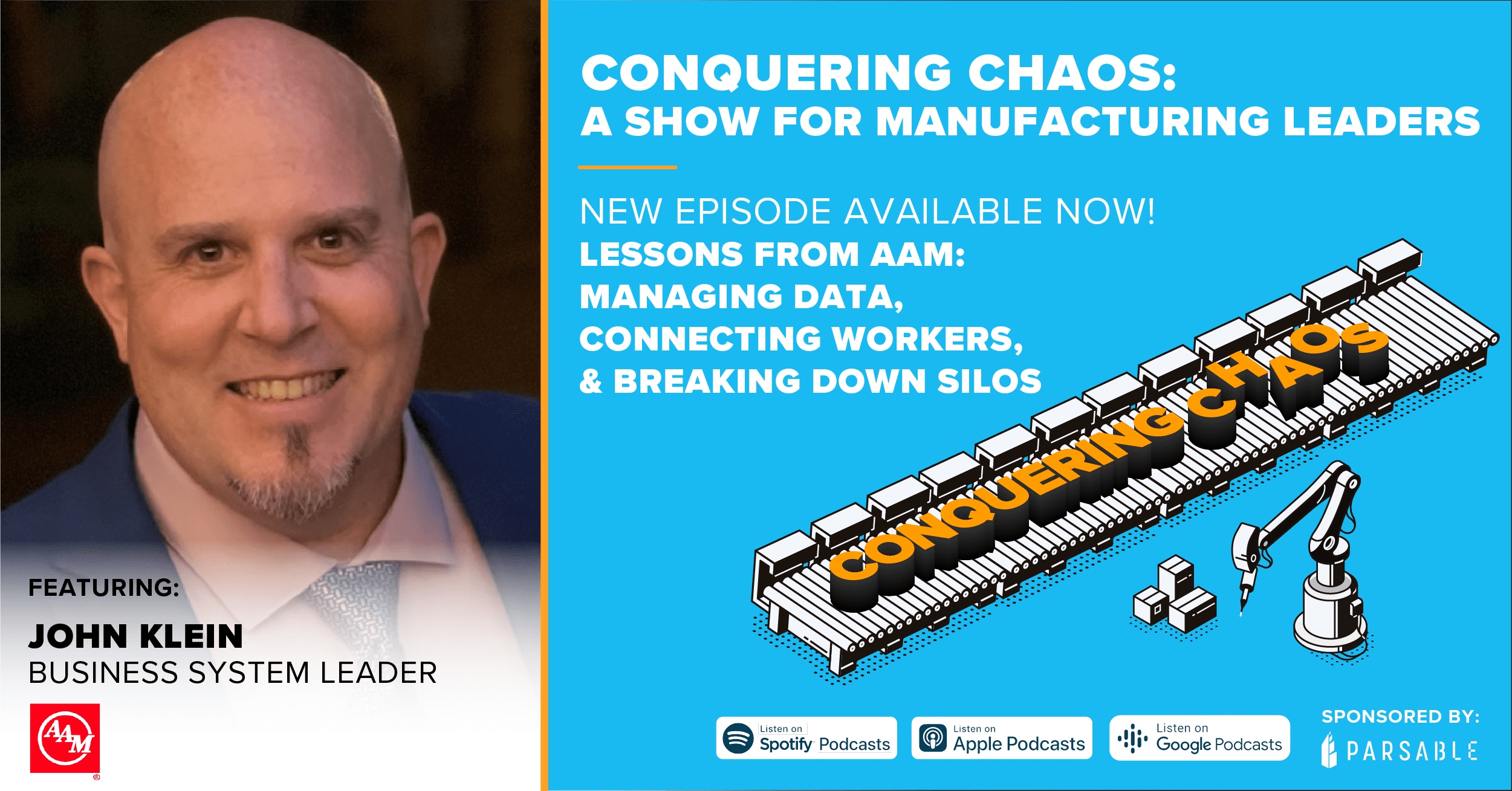
One of the most compelling reasons to digitize your operations is the access you get to new data points that were previously uncapturable. New data points bring new visibility. New visibility brings new insight. And new insight brings new innovation.
But what data points are needed? And are these insights reserved for certain roles? Or can data be a powerful in-the-moment companion to everyone within manufacturing operations? Today’s guest, John Klein, Business System Leader at American Axle and Manufacturing joined to give insight on how new data points combined with powerful visualizations and connected worker technology has enabled people at all levels within their operations to drive innovations.
Join as we discuss the:
- Power of frontline activity data
- Importance of custom dashboards for data visualization
- Challenges of data collecting through paper
- New way of connected work for frontline manufacturing operations
- Way to scale with frontline analytics
___________________________________________________________________________________
Experiencing disruption on the frontline? We can help. Request a demo today.

___________________________________________________________________________________
Check out the full episode below:
Josh: To everyone tuning in right now, thank you for joining us for this episode of Conquering Chaos. Today, we’re chatting with an accomplished manufacturing leader on the impact you can have on your manufacturing operations with the right Master Data Management strategy. We’ll also talk about the obstacles you might encounter on your master data management journey and what you can do about them. You’ll hear about the ups and downs, expectations and realities, obstacles, and outcomes of our guest’s experience achieving large-scale data collaboration through Master Data Management.
Now, speaking of our next guest, he’s developed quite the expertise on tapping into and leveraging the power of data across many different aspects of manufacturing through a decade of firsthand experience. In fact, in recent years, he’s helped his team deploy close to 150 large-scale dashboards, leveraging data from Parsable, who’s the sponsor of the show.
As the business system leader for manufacturing systems and data analytics team at American Axle and Manufacturing, he’s supporting all industry 4.0 initiatives, including Connected Worker, which is also the deployment of Parsable, and Master Data Management from the business side of AAM for all manufacturing facilities and test prototype centers worldwide. Please welcome to the show, John Klein. John, thanks so much for being here today.
John: Thanks, Josh, for that warm welcome today. It’s much appreciated,
Josh: Well deserved. I’ve enjoyed the conversations that we’ve had together and I’m excited to share some of the insights you’ve previously shared with me with the listeners of the show. We’ve covered a variety of topics on the show in the past that highlight how essential, how– All right, Bill, here’s our first edit. We’ve covered a variety of topics on the show in the past that highlight how essential data is to the future of industrial operations.
Data can be a means of enabling digital transformation, industry 4.0, artificial intelligence and machine learning, digital traceability, and more. All of those buzzwords aside, there’s power in the data that can be realized independent of broader transformation initiatives, which means that by tapping into and effectively using this data, you can make improvements sooner rather than later. John’s got firsthand experience with this topic, and that’s what we’re going to be getting into. John, I’d love if you could talk to us about your experience working with data within manufacturing operations and manufacturing systems
John: Absolutely, Josh. It’s been a long journey, 27 years for American Axle in manufacturing of data collected in many different fashions. A lot of the data that’s collected from the assembly lines is automated to a certain extent but a lot of data is not. There’s a lot of manual collection as well. There are a few different aspects of how we collect data, whether it’s from the operators, we look at who’s the consumer of that data? Is it management, is it a coordinator, a VP? That all requires different levels of visualizations for that type of data. In our 27-year period here, we’ve collected so many different variations and a lot of it has just been sitting idle.
Now there are pieces that we utilize but a lot of that transformation of data has been always a manual process. Someone has to download it or they have to go out to a machine and put a memory stick in and export that and do a regression on an excel sheet or whatever transformation tool that they’re using.
Now we’re getting to the point where we want that data, the insights of that data, that rich media, that is involved with that in the person’s face at the click of a mouse. There’s so much value to that visualization. Taking the next step in that is going to be the predictiveness of it. I think we’re going to get into it a little bit, a lot of the machine learning and the AI portion of this we can be very, very punctual with what happens by learning what a machine is doing.
Josh: I think that is such a great description of what we see in across many different manufacturers. Like you said the years may vary, but data’s been collected for a significant amount of time, in some cases, the collection of that data is automated. In a lot of cases, especially when we’re looking at frontline activity and operations, a lot of that data is collected manually and then transformed manually, and then analyzed manually.
I think some of the things that you’re setting up when we start talking about these advanced solutions, whether it’s artificial intelligence, predictive maintenance or prescriptive maintenance, and what that actually means, you really have to have this streamlined, consistent, effective automation of data collection in order to effectively implement those programs. When you think about the future and the vision that American Axle Manufacturing has for the use of data within day-to-day operations, what does that look like?
John: The future of data within American Axle is a complete collaboration of all the legacy system’s data interacting with each other in a manner to do just what was described. Be predictive, be prescriptive, make decisions. There still has to be human interaction. I want to get that very, very clear that someone has to be looking at the data. They have to still utilize the visualization to make decisions.
Now, machine learning can be a very good tool. Still, you have responsibilities, you have accountabilities that are still present. Running your day-to-day operations off of straight input data, yes, it can be done, but there are cautions around that. Someone still has to be monitoring that to make sure the accuracy is there and we’re delivering exactly what we want to the consumer.
Josh: I think that’s such a great point that at no point are we talking about eliminating people from the equation. We’re talking about freeing up some of the– I wouldn’t say responsibility, some of the burden that gets placed on a lot of individuals to go and collect this information, take it, transfer it into another system format whatever that is, and then do something with it.
To your point, even when artificial intelligence does get implemented, AI is not at the point of making decisions on behalf of people. It can present options, it can identify trends and opportunities, but to your point, John, and I completely agree, and we’ve had previous guests on talking about AI, humans decide. You have to have the people who have that understanding to make the call.
John: 100%, Josh. I think that utilizing that data to remove the non-value-added work is essential. By removing that or taking and shifting that allows us to free up time for let’s say your quality engineer for them to do something more engaging on their aspect that they can go out and do a hand inspection if necessary. Whereas before, if they were analyzing the data, maybe that portion of it got skipped for the day. Not saying that happens, but you’re re-appropriating time in a more valuable manner.
Josh: I think that’s such a great way to think about it, really invoking that idea of non-value added work that’s prevalent throughout a significant portion of operations that I’ve seen firsthand, that I hear from guests like yourself. I love that idea of there’s something more valuable that you can be doing with that time. I’ve talked with the quality managers, for example, this was in a health and beauty manufacturer who would spend almost up to two hours per day just preparing a report that they would then talk with their overall management committee.
The reason it took that long is because they had to go almost line by line to find out about specific items, talk with people, get that information, take it back, compile it into the format that’s required, and then share that with individuals. I think you’re absolutely right. There are a lot more value-added activities that can be done during that time.
John: Thinking about it from the top-down approach, at least on the American Axle side, when we were looking at a quality report just for quarterly-based requirements, not to go too far into specifics, but we had certain data pieces that reports that had to be put together by individuals within a plant, and that report had to be either emailed or the data had to be compiled on the backend throughout the quarter.
Now with the use of Parsable and analytics tools like Power BI and the collaboration between the two of them, now that report is live. We’re taking all of that data that’s being ingested, we’re collaborated with Workday within the same transaction, that allows us to give that minute-by-minute view if necessary of what is going on with that particular report. We cut out all of that reporting activities that were pulling down and compiling that non-value-added work and supporting what we had just talked about.
Josh: I think that is such a great call out, and I am so excited with how AAM and the team there has found the ability to really work towards these goals to work to eliminate some of these non-value added activities, automate that data, collection of data that previously was inaccessible and really set such a strong standard for what the future is going to look like because let’s be clear, you want to talk about like what digital transformation Industry 4.0, all that stuff actually looks like for manifesting. What it looks like is each person involved has access to the information they need right when they need it so that they can make the right decisions or take the right action.
What you’re describing is exactly that. How do we get the data, automate it to a visual system in a way that they can interpret it, and then with that interpretation take action? That when we start thinking about some of the difficulties manufacturing’s having in general, that becomes very powerful from that ability to adapt and adapt quickly because now you’re getting the information you need, you can respond, you can see the impact of that response, and the chain effect just keeps going.
John: That’s correct. It takes down the silos of different groups within a company. There’s a lot of different groups that keep American Axle moving on a day-to-day. Most of the time, quality’s information is quality manufacturing, engineering’s information is manufacturing engineering’s. There’s a lot of silos and walls put up and in the best interest of MDM, you’re taking all of those collaborations– procurement, that’s another big one. You’re taking those silos down and collaborating the data together to perform your day-to-day in a more efficient manner.
Josh: I love that you bring up the topic of silos. I want to define for our audience, you mentioned MDM, that’s Master Data Management. I’d love for you to tell us what exactly is master data management and why it’s so important.
John: Speaking of silos, you have all the data that is collected from our product engineering team, from procurement, from quality, maintenance and so on and so forth. What we do, or what the intent is on the master data management side is to collaborate all that data. We take large-scale portions or the data as a whole and things that are common. I’ll use machine numbers as an example.
A specific machine number 1, 2, 3, 4, 5, 6 is the same identifier in every system that it touches. When someone is talking about it in the maintenance system or they need to collaborate with it within the maintenance system and connect that to quality, when we put that into a tool an MDM tool or an analytics tool, we know that that machine is the same, or that part number is the same as it carries across AAM, does not matter which system it touches and it allows us to streamline those processes.
When you look that machine up, or you want to know a cost on that machine or how it impacts the business or the collaboration between two different systems, we can make that relationship very, very quickly. The big portion of MDM, I want to take a step back, two of the major silos that have always been in place is IT and the business side. Doesn’t matter which company you work for. There’s always been a little wall between the two.
With MDM and our group’s relationship, we’ve brought that wall down, and we do a very, very good collaboration between IT and the business side because those two have to work in unison in order to make this successful. There is a lot of information. There is a lot of different reporting pieces that need to happen to make this a very, very successful operation.
Josh: I think that was a great example. I like to think of it. This is how I’ve interpreted. Maybe for those listening to this will resonate with you. Whenever we’re trying to understand, why are we getting the results that we’re getting? John, to your point, oftentimes that data it’s coming from a ton of different systems. It’s almost like you’re the detective and the production, the operation that’s the crime scene, and you’ve got different witnesses who are essentially describing the same event. It might be I saw a car, it was – blue and someone else says, I saw a hatchback, it was a light sky color.
We’re all talking about the same thing, but it’s not quite matching up. You see it like in systems where you’ve got asset number versus machine number versus maybe it’s just a general line number, but it’s all pieces of it.
When you’re looking at the Master Data Management what does it take to actually bring that data together, is the expectation that each of these different systems, their identifiers not just the machine number, but the way that they classify some of the data that they’re capturing matches, or is there a need for something in between all of that’s essentially converting or translating the data that’s coming from those systems?
John: Yes, depending on how the deployment or the decision is made between whether it’s American Axle or another manufacturing company or any company in general, you either can utilize tools that you have or you can pull data into a giant data warehouse in a large scale S3, some sort of cloud-based location, or you can utilize a tool that is a third party that allows– much like Parsable, it does matching and the collaboration between the data systems for you and there is a little bit of structure that has to go behind it.
With that being said, the end result is typically the same. It just depends on resources, how fast you want to get there. An off-the-shelf tool may not deliver everything that you’re asking for because your business practices may be a little bit different. It’s not a cookie-cutter approach and I think ours is going to be a collaboration of the two between data warehouse interaction as well as a third-party tool to assist.
Josh: That’s a good point that comes up pretty consistently. With all of these lessons that we’ve shared, there’s always the caveat of the situation that our guest was in. Their environment is fundamentally different than yours. It could be the exact same industry similar manufacturing processes, but there’s a ton of different variables that always lead to the situation where that you have to decide what’s going to be best for you and to your point, you’ve got a bit of a mix and match approach. For some situations it’s expected to be standardized in this one system and in other situations you’re having to use a third-party tool to bring it all together and that’s okay.
John: That’s correct. There’s a lot of data out there that formatting for a lot of different formats that data’s plugged in. Different systems use different extractions, and I think that that’s where that third-party tool comes into play. Could we get there? Yes, doing it on our own. It is possible, but how much time and resources are we going to utilize in order to make that happen? It all comes at a cost. It’s all time it’s all money.
Josh: To your earlier point, is that time better spent elsewhere?
John: That’s correct.
Josh: Such a good point. Are there any sources of data that you found to be the most critical or do you think this is going to be something that changes depending on the problem you’re trying to solve?
John: Yes, absolutely. Product data and procurement, those are two big ones. Our product engineering part numbers, cost of materials, direct, indirect, those are all big key players in this. When we look at this MDM portion or MDM journey, there are ancillary costs as well. Essential, but they are ancillary to that. Our business is based of production. You still need maintenance, you still need testing.
You have a lot of those secondary costs that are essential but get sidelined, but they are still there and required but the big key player is production. Bill of materials, how do we collaborate that all together? I do believe our last analysis of data for one axle build, we have over almost 13,000 data points that we’re pulling off of one bill. It’s more than that on certain things, but that’s just a round number. That’s significant. That’s one axle as it moves down the assembly line. Sheer production numbers, we have millions upon millions of data points that we are taking daily. I think production is going to be the biggest player in this.
Josh: I love exploring this concept like that. You brought up 13,000 data points for just one build, leading to millions when you look at all the things that you’re producing, especially when you’re starting to think of not just one particular location or one particular line, you’re talking across the entire operation of the company. That’s a ton of different data points.
What I love exploring with the customers that I’ve been able to work with is this idea that there are variables that are impacting the operational results and to some of the earlier points that you made, if you’re not able to collect or access those data points, you’re not going to be able to really contextualize why are we getting the results that we’re getting? You may have some idea, you may be able to draw some conclusions, but those more specific data points you can have, the more accurate you can be assured of your analysis, and then of the action you take in response to your analysis. This is such a great topic.
John: That’s correct.
Josh: When you think about who benefits from access to these data points, this data pursuit is this really for leadership or is there something in it for everyone?
John: All of this is cross-functional. I think one of the original statements that was made was, it doesn’t matter if you are a production supervisor or a VP, you are a consumer of this data in some manner. The visualization may be different, but the data collection and the projection, whether it is part counts or part costs, it’s all relative to what level you need to be viewing this data at.
That’s how we build our analytics. A lot of these dashboards that we talk about are built for specific groups but at different levels. They have different filtering options so that maybe I just want to know what the daily build number is. That’s it. As a production supervisor, I need to know what my hourly part count is. I need to know how many parts were going through each operation at a given time. Am I meeting my tack? Am I below? All these different data points. Now, when you get into the higher levels of management, unless there is a true problem, you don’t really need to know that. You’re focused on what is the number.
I think taking all of these data points and putting them together, allows us to make better decisions. That does not matter what level you’re at. As a production supervisor, you may see that your part counts are low, and you may need to go address something, and previously, you weren’t able to do that if it was manually counted. Now, we have advancements in technology that allow us to pull that data live. Well, as close to live as possible so that you can make a well-informed decision.
Josh: That’s such a key point. There’s something in it for everyone. Really, the power is not the data telling you what to do because back to your previous point, it’s still up to the individual with the expertise and the knowledge to make the decision, but that data helps us make those informed decisions faster across all levels, whether it’s needing to intervene on how a particular asset is performing to what is a strategic investment that we need to make in order to further get us towards our goal? I think that was such a great call out of its collectively making better decisions. Now, one thing that we’ve seen– Oh, go ahead.
John: No. I was just going to say we talked about predictive maintenance, prescriptive, predictive, on that token, talking about it from a production supervisor standpoint, you’re collecting all that machine data. If they start to see temperatures go up on a certain bearing or something like that, that data was the ultimate or the predecessor to this was failure. It was a failure or dictated a response from maintenance.
Now, collecting this data and enabling machines with different types of sensors and collecting data on them directly, you can make that decision that when a bearing gets to this temperature, it may be going bad or you may need to adjust something or something to that effect. All that data compiling and being able to utilize it drives that effort to be more efficient.
Josh: Absolutely. Everything from taking action to getting to the root causes. With your bearing example like you said, you can see that after a certain amount of time or certain changes in what’s being observed that can then start to lead to the understanding that oh, we need to take action in order to prevent a very costly downtime situation. You could even take that further, depending on the data points that you’re gathering to, say, bearings that we get from this supplier, versus others and that type of comparison so that you can optimize, share that data with your suppliers so that then they can take action and improve their products. Now it’s going to be a longer time in between those potential failures.
John: Yes.
Josh: One thing that we’ve seen to be consistently true here at Parsable is that, you mentioned this earlier, is that there’s a big gap in getting the data in some cases, especially from frontline operations. Most operations that we start out working with have difficulties with data collection and sharing. A big reason for that is relying on tools that cannot facilitate the level of data capture that’s needed to understand what’s happening, when, how, its impact, et cetera.
In the ways that they typically are capturing that data, it’s still incredibly difficult to access or it requires significant processing to make it usable. What I’d love to hear from you, John, is what’s one of the biggest obstacles manufacturers may encounter when attempting to build a culture of analytics and data collaboration?
John: That’s a great question, Josh. There are many challenges that we face in this arena. One of the things that I think that is a key point is talking to who is consuming the data and understanding what their requirements are in order to provide proper visualizations. One of the misconceptions that we falter at it this is one of the lower points for us, and this goes for any type of development. If you develop in a vacuum or it’s a singular person’s idea and you take it to the floor, it may not be well received. You may not be delivering what the end user needs. I’ll take it a step further. You may be over-delivering on the data that you’re projecting.
I think that that’s one of the failures that we’ve seen is from our perspective, we’ve developed dashboards that support different types of data collection and operations, but on that token, we were over-delivering certain pieces of data and requiring, I’ll use the supervisor as an example again, requiring them to do more than be concerned about what their day-to-day operations are. To be more focused on the data rather than looking at the data quickly and then making an educated decision, not interacting with the data, entering more data although that’s not the idea. The idea is to be more efficient in what you’re doing.
Josh: Such a great point. Data is not the goal. Data should support you in accomplishing the goal, but-
John: That’s correct.
Josh: -putting that first, that’s not what needs to happen. I think that’s a great example that you also brought up because we talked about there’s something in data for everyone, however, that visualization, that ability to be able to interpret that data has to be specific to the consumers. It’s almost like you’re having to build your own product for each individual to use for their purposes to serve their pursuits of that goal.
John: Yes, absolutely, and that can be very specific to groups. Whether it’s quality or manufacturing or testing, it doesn’t matter. It could be generalized auditing, right? All of it is all reliant on who needs to consume that data and at what rate, what is the frequency of that data?
Josh: Now, one thing that I’ve seen with a lot of manufacturers that I’ve worked with is that in some cases, this ability to automate the collection of that data or accessing the data that is captured is very difficult and time-consuming next to impossible when paper routines have been the norm for decades. I’d love to hear from your perspective, is paper problematic, is it outdated or is it still an effective way of collecting data?
John: I think this is something that we’ve discussed in the past is, no paper will never go away. Paper serves its purpose. It’s been there forever. It plays a key role in that. I don’t think I can type on a keyboard faster than I can write on a piece of paper, but what that piece of paper lacks in delivering data, you make up for in punching the discrepancy in time that you have punching the data in. We can fill up file cabinets and storage units with paper processes and all of that, but it’s stagnant. It’s data that’s just sitting there that someone needs to do something with.
Now, on the industry 4.0 journey, working with the connected worker platform of Parsable, we’ve been able to collect that frontline data work whether it’s audits or torque data, or any type of manual transaction that was done hourly part checks are a big one, stuff that was done on paper previously, we can do that digitally. Now, does it take a little bit longer?
Yes, it does, but again, you’re making up for it in what you are projecting in the analytics tools, in the collection of data, because if there’s ever any type of audit finding or anything that needs a deeper dive needs to happen in on the paper process, you may have 5, 6, 7, 8, 10 people looking for one audit that was done three years ago. Whereas on the digital transformation side, I’m three clicks away. As long as my computer’s on, I can typically find what I need in a matter of seconds, not hours. I think that shines the light on that type of it, but paper still plays an essential role. I don’t think you’re ever going to get away from that.
Josh: You mentioned, yes, it may take longer, what you’re referring to versus a digital program is just that since growing up, most of us, the next generation may be a little bit different, not Generation Z, but like generation Alpha I think is the next generation coming up. Maybe that’s different. Maybe they’re not using paper as much, but growing up I know, everything I did, whether it’s homework, papers, et cetera, note taking is very paper-based.
I have an iPad that has a digital sheet of paper that I use to take notes on because I prefer to write it out. There are some things I absolutely agree with you, John. There’s a convenience, there’s a habit in our personal lives of still using paper in that functionality. When you say it takes longer, is it just the typing it in versus writing it down, or are there other components to that?
John: That’s right. If you are not directly ingesting data, you still have to find the job in your workflow, find the appropriate section that you need to be in, make sure that it’s completed, type in the numbers if we’re using numeric fields, make sure the task is complete. There’s a lot that goes into it, whereas if I was just writing 10 on a piece of paper, it’s very, very quick. I don’t have to log into a piece of paper, but again, that paper is static. It cannot deliver what typing that data in does.
Josh: Like you mentioned, one of the benefits of that digital approach being three clicks and you’re audit-proof, that sound dramatic. You got to still got to make sure you’re doing the right things, but sometimes– I’ve worked with manufacturers who are getting penalized only because they couldn’t prove that they did what they were supposed to do. They did what they were supposed to do, but they couldn’t prove it.
John: That’s right. Well, I think of it we’re looking at different pieces of this, searching for that data, maybe you’re under a time crunch and auditors there and you need to provide what they need right on the spot. Sometimes that’s not the easiest thing to do because I’m going to be honest, in an audit scenario– I’ll use internal audit, if they don’t find what they need, they’re going to keep digging and digging and digging, and digging, which that’s not a bad thing, but your data should be there at their fingertips. It should be a quick response when they ask for it.
It’s being prepared for class. You’re always told to be prepared for class and oftentimes, it’s the teacher throws a pop quiz at you, well, are you prepared for it? Did you learn from your mistakes or did you learn what you was taught over the week? Well, maybe it did and that shows in the quiz. Maybe you didn’t. That’s how an audit goes.
If it’s on paper and I have to fiddle with finding it in a file cabinet, that’s not the ideal scenario. Whereas if you can say, “Hold on one minute”, and they see the same screen that you’re looking at on an overhead or a display or you’re sharing screens and you can share with them, “Here’s your data that you’re looking for” in those three clicks, two clicks, one click, whatever it may be, they will appreciate that more. It brings to light that you are doing exactly what the auditor is asking or you’re well within compliance.
Josh: That traceability, that visibility, that quick access, it does lead to a totally different experience. Now, you mentioned some difficulties you had and that you’ve seen in order to access that data, you have to search for it, find it. It’s stored somewhere. Other people are looking for it. Someone may have it. Maybe it didn’t get turned in. What are some other difficulties aside from finding data that you need to access? What are some of the other difficulties you’ve experienced firsthand as a result of relying on paper to capture and transfer information?
John: Yes, another great question. I’m going to go into the testing facilities. One of the things that before Parsable and digital collection, all of the test data was stored in binders. A test can run from minutes to months, days, years. That binder can get very, very thick, and people will flip through pages. Now, we’re all human, and if you have greasy hands or we spill oil on the paper, that log book of all the transactions that were written down, oil can do some nasty things with it. You could essentially erase months and months worth of testing, logging. You still have machine collection data.
The accountability side of it, you don’t have it anymore, because the book got soaked in oil, or it has a greasy handprint on it, or it fell on the floor and now it’s soaked in water. We’re all human, and error is going to happen at some point. We all want to be as perfect as possible. When you’re trying to be efficient usually, or you’re racing around, that’s when the issues happen. Right? Then try to get the data out. That’s the other thing is, if I’m looking for a quick log and I can’t find it, because that page got ripped out of the binder and fell on the floor, and now it’s under the desk or something to that effect, now that we’ve transformed that facility into 100% paperless, they can find the test order very, very quickly. They can go to it and see exactly where that transaction took place.
Josh: That’s a big accomplishment. 100% paperless, that’s a huge undergoing, because like we talked about earlier in the conversation, this has been the norm for a significant amount of time. How did your team tackle that? How did they accomplish this?
John: Yes, that right there was working with Parsable in general. Our journey began June 3rd of 2019. In that journey, one of the goals that was set top-down approach was that our testing facility, our Rochester Hills testing facility needed to be 100% paperless by January 1st of 2020. That’s six months. In that time period, we had a great team of not only Parsable representative but American Axle Associates as well. We put together that team of engineers and technicians in order to achieve that goal. With the Director sponsorship that failure is not an option. We will be digitally proficient and paperless within six months. Myself championing that project.
It had its challenges because we were so reliant on paper that you had to break the mold. Did it come with its share of failures? Yes, but fail quickly, because you want to get that out of the way and make sure that not only that Parsable gets their stuff in order, but also American Axle so that we can be successful together. I can tell you that through the ups and downs, we hit that 2020 marker and by January 2nd, all the paper was gone out of the facility. There was a lot of organic growth within this. We use the tool primarily for job instructions or work orders testing, but that organic growth turned into audits.
What else can we do with the digital tool to collect data? How else can we remove different types of paper? Fork truck inspections, very, very simplistic stuff that you would think people just do day to day. We transformed it into the digital tool and now we’re able to collect metrics on it. How many fork truck inspections were done in a given period for a given truck? You can see that now, whereas before you didn’t have that luxury.
Josh: Now with implementing any type of technology, changing it’s always difficult, especially the bigger your organization is. What resistance to change did you encounter?
John: Yes, sure. I think we talked about it already. You have the up-and-coming workforce that they don’t take too kindly to paper, because they’ve never utilized it in a capacity that we do. The up-and-coming workforce, they’ve always had a cell phone in their hand or a laptop in front of them, a tablet to take notes on, and we need to embrace that. Those are some of the challenges with the older workforce, is they’re willing to learn. A lot of the resistance on that was someone’s going to have to teach us how to do this. Through proper training and different classes, training sessions and job aids that we put together, we tried to make it as simplistic as possible for everybody.
It doesn’t matter the user, if you have a task to do, you pick up the tablet. We use an audit as an example. Doesn’t matter if I’m logging in or you’re logging in. It’s the same. If I have to work on that line that day or that machine that day and I need to perform an audit, I can step through it. Top to bottom. It’s the same for everybody. That helps us in the manufacturing arena too.
If someone is covering overtime for another associate, that day-to-day hourly part check is always the same. If I haven’t done that in six months, but I’m still qualified and trained for it, I step right in, I log in and I go and it’s muscle memory for the brain, right? You know that I can log in and see the same thing no matter where I’m at.
Josh: That’s great to hear that. Really, with some of the resistance, it was more so on. I just need you to make sure that you help me understand how I can do this and how, as a group, you can provide something that’s simple and consistent. Then, like you mentioned, you saw, as a result of your efforts that organic nature of growth. With that idea of that growth, that organic growth, was it a situation where as people were exposed to, okay, I do it this way for this activity, was it a situation where people were starting to suggest things that they would like to do in a similar pattern? How did that really come about?
John: Yes, it was people analyzing their day-to-day operations and bringing topics to us and saying that, ” Hey, we have this process. Can you author a quick template to see how that would look?” In the beginning, there were ups and downs to that, because we were getting used to the tool. We were trying to learn what the most efficient manner was for us to teach the tool to people and organize the structure.
That’s why we put together a group, at least in the tech center, that helped us provide that structure. On the manufacturing side, same type of resistance. Everybody was very, very new to this, and then we started to build some structure around it, and now we’re accelerating very, very quickly with global deployments in every single country that American Axle touches, Parsable is a part of in some manner, the connected worker aspect, from supply chain, quality, manufacturing. It’s touching just about everything right now, which is it’s been very, very challenging up to this point, but we’re more comfortable at where we’re at now than we were let’s say a year ago today.
Josh: As you go, you learn, you grow, strategy gets to hone in, and more people get bought in.
John: Absolutely. It’s always iterations and continuous improvement. You need to audit yourself just as much as you need to audit others.
Josh: That’s such a great call out. Auditing yourself, looking at are we doing things the way that is optimal or are we doing things the way we’re doing them because we were limited previously. That takes a lot of rethinking and challenging of how you’re doing things.
John: Yes. I think we’re doing this to improve upon processes that are in place. What that means is if we’re expected to do 10 things, can we get all 10 of those things done in one day? Maybe, but are they done efficiently or are we just going through the motions? At this point with collecting all this data no matter where it’s coming from, we can highlight those inefficiencies. We can take and draft conclusions that maybe we don’t have enough people in one area, but we’re overstaffed in another area, and that certainly helps to be more efficient and utilize the tools for what they’re there for.
Josh: I think that the other thing that you called out, particularly on that organic growth side, is that the more you can get people auditing and analyzing their day-to-day like the example that you gave of do we need this many workers here? Is there a way that we can shift it? That’s someone at a certain level doing that. There a different perspective of saying here’s an activity that I consistently do that I think we can do better leveraging this tool. That type of ownership by individuals, that ownership in that empowerment, that is such a key factor for success.
We had Tom Shorma who’s a former CEO of WCCO Belting who talked about how they increased throughput by 20%, and the way that they did that was really just by listening to their employees, taking their ideas, implementing those ideas and running with them. That built a culture of continuous improvement, and ownership, and empowerment, that actually led to increased retention rates of their employees as well as easier time recruiting employees, because they had so many referrals internally. Some of these lessons that you’re talking about there’s better or bigger impacts that are sometimes hard to quantify, but they touch so many different parts of the operation.
John: 100%. When you’re listening to the boots on the ground of, it shows that you’re in tune with their needs, and you’re listening and responding to what they’re bringing to the table. We’ve been in scenarios where again developing in a vacuum and not understanding the full process ourselves, then you take it out to the floor and you run through it with an operator or an engineer and they say well we don’t do it that way.
We do it like this. If you move this step around and change this or make this little edit here, you’re going to get the process down 100%. We learned that in paper processes too. We’ve had, I’ll be very generic, but a delivery route. You have to 50 different locations in the Excel sheet that they printed off and they would select or check off that they made it there and they delivered X amount of pieces, or quantities, of something. It was they started at the top of the paper, and then they went to the middle, and then they went back to the top, and then they went to the bottom.
Well, we sat with that driver and said can you label your route for us, top to bottom? We digitized it, and we put it in there, and now that job is more efficient, because as they to their station, they’re clicking through the steps within Parsable to plug in the data. It’s little wins. The small gains will provide large-scale efficiencies in the end.
Josh: Well said. That is absolutely true. The more you can focus on those little day-to-day moment-by-moment wins, that’s what’s going to build up and have that impact. The team at AAM has done an incredible job of finding these ways to make life just a little bit easier for workers at all levels. I’d love to hear from you John, when you think about your experience and your efforts around master data management, connecting workers, moving away from paper, what are you proud of your team for having accomplished?
John: One thing that we highlighted was on the technical center, them being able to shift over to a fully paperless facility within six months, that is a huge accomplishment. On the manufacturing side, I think the collaboration of our quality department, working with them to streamline processes that are globally deployable in an expedited manner has been second to none. I encourage everybody that’s out there to evaluate how you can scale your approach, taking one process at a time in trying to standardize that process.
We learn very quickly that the silos really caught up, because plants operate as essentially their own entity, but when you’re cross-functionally you can learn from different. One plant may have a really great idea for the same thing that another plant is doing, and you can build upon that. That way you can take an audit and make it standardized across the globe, and deploy it in every single language very, very quickly, and then draft analytics out of it it. It has huge returns. That’s a huge accomplishment for American Axle from an auditing perspective. I’d like to give credit to the analytics team.
We do a lot of crunching of data and with that being said that we’re being very, very receptive to the business side owning a lot or collaborating with IT to make these analytics and visualizations, what the owner wants or what the consumer wants, and it’s done very, very quickly. We scale so fast on the analytics side that’s almost scary that we can deliver in that type of manner. It used to be that a dashboard took months and months to or a singular report took months and months to put together now, what was taking months is now taking weeks or sometimes days because it’s a quick iteration.
Josh: Yes. When I think about all the different topics that we’ve covered today, and I think about some takeaways that I want to make sure our listeners who maybe haven’t yet started to move away from paper or established a solid master data management program or haven’t explored how they can connect to their workers. Some of the themes that I’m hearing from you John, and I’d love for you to add on to them is this idea of whatever you’re building build for the user.
We talked a lot about the data and visualizations being something that is specific and built in collaboration with them so that it perfectly serves their needs, and helps them accomplish the goals that they are tasked with, with working towards. I love what you just called out. Standardize worldwide. Start with one process, try to standardize that, get what’s working from all these different plants, make that thing that works globally and that helps with that collection of the data, and really try to drive that standardization. Then the other element that came up was focusing on those little wins. It’s not necessarily about the big wins. It’s about those tiny moment-by-moment, process-by-process, day-by-day activities and wins that can lead to those big results. Are there other pieces of advice that you would give to our listeners who are not as far in their journey as you are?
John: Harnessing the talking to the frontline worker, understanding the process, what they go through. If your target is that area, you have to learn what they do. That goes for any type of process that you’re trying to digitize, whether it’s a quality process, or a manufacturing process, or a testing process, take the time to learn that process. Now, you don’t have to go far into the weeds on what that process is but educate yourself on it.
Learn what they do day-to-day, it will only help you in making that digital transformation. It will help you in creating those data visualizations for them because the end goal is to be more efficient in everything, whether it’s collecting large-scale data and providing insights to it, or providing a simple audit that’s digitized. I think listening to the person or the consumer that is affected by that data or keying in the data, that collaboration being the middleman on that, you need to know both sides of it, the consumer and the inputter.
Josh: Great call out. Great call out and ties into that theme of really making sure you are serving these different groups and not necessarily dictating towards these different groups. Such a key call-out, helps with adoption as well, because people are going to resist things that they didn’t necessarily have a hand in helping build– I think those are great.
John: That’s right. They need to feel involved.
Josh: Yes, because you don’t want this to be a siloed project.
John: That’s good.
Josh: I think this has been a great conversation, John. I feel like I’ve learned a lot from you in our time together in just about any conversation that you and I get to have together. I’d love for you to share with us how our listeners could continue the conversation with you if they have questions or just love hearing from you and want to connect.
John: If you’re interested in American Axle & Manufacturing, you can find us at www.aam.com to learn more about American Axle as a global tier-one supplier for automotive.
With that, thanks again, Josh. This has been a great podcast experience.
Josh: I appreciate that. All right, John. Thank you.
Listen to find out how industry leading manufacturers use Parsable to get new data, connect workers, and break down silos!