Faster, Better, Cheaper: How Machine Learning & AI Improve Manufacturing
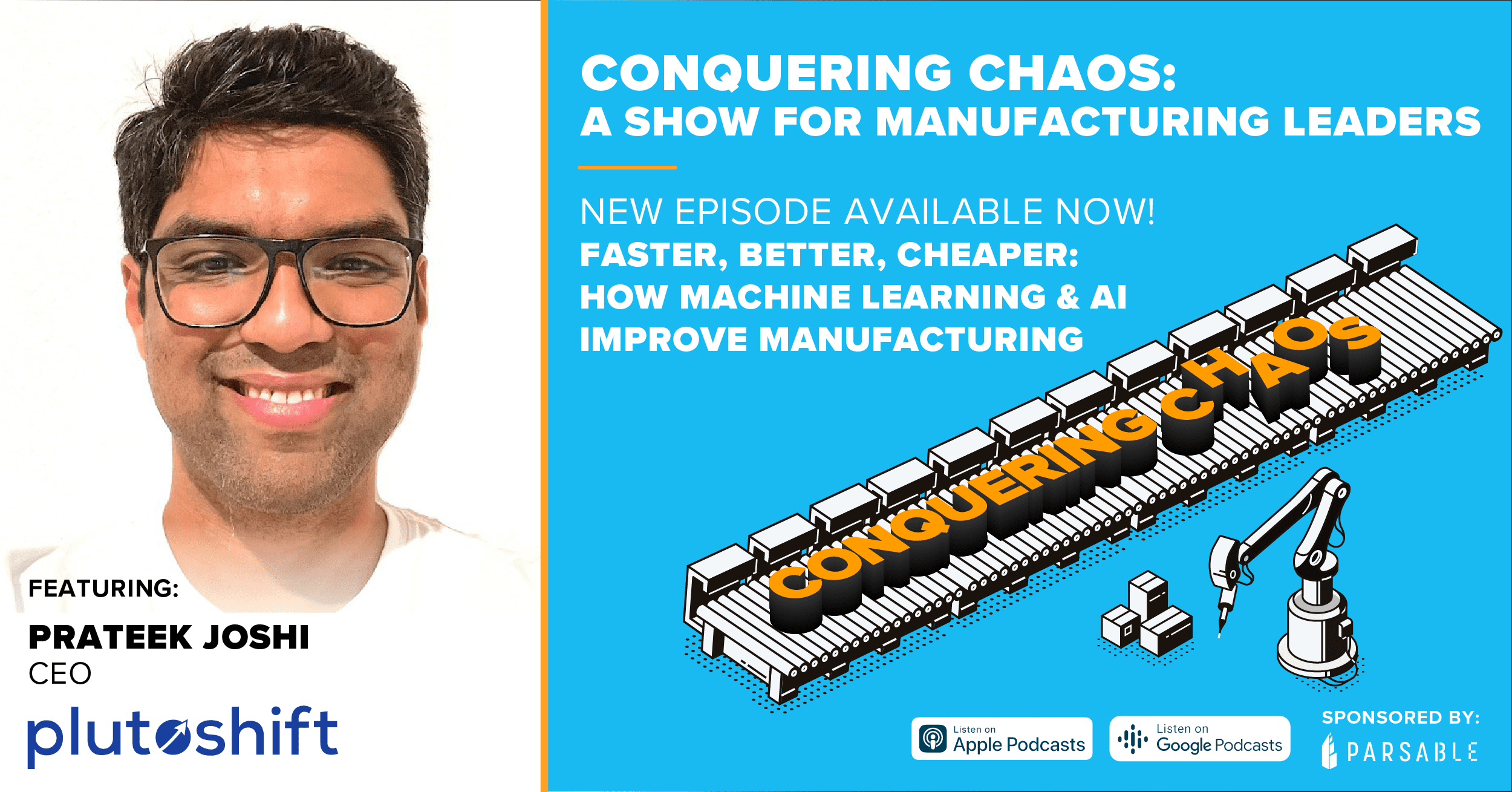
Machine learning and AI are critical to the future of manufacturing operations.
To get you up to speed on the topic, we spend this episode explaining how they work and showcasing the incredible ways in which industrial companies are implementing them into workflows.
And we’ve got the perfect guide: Prateek Joshi, CEO of Plutoshift.
A machine learning expert, he has written 13 books on the subject. Today, he’s bringing AI to industrial organizations around the world via a data platform that monitors physical infrastructure.
We discuss:
- What AI is and how it works
- How to get started with AI
- AI use cases in manufacturing
- Why Prateek transitioned from tech to focusing on industrial organizations
___________________________________________________________________________
Are you ready to start your digital transformation journey? Request a demo today.

________________________________________________________________________________________________
Check out the full episode below:
00:00:00] Prateek Joshi: You have a certain task that a human is doing, and they can continue to do it, but by infusing AI, they can do it a whole lot faster with zero errors.
[00:00:12] Josh Santo: Welcome to Conquering Chaos, the show for manufacturing leaders. In each episode, we are connecting you to the manufacturing leaders of today who are driving the innovations needed to future-proof the operations of tomorrow. If you feel like your time is spent fighting fires and trying to control the everyday chaos, this show is the show for you. My name is Josh Santo. I’ll be your host. Welcome back, Conquerors of Chaos, and listen up. We have an insightful episode in store for you.
Our next guest has a background, unlike our previous guest. He started his career in tech, working for major innovators like Invidia and Microsoft research. Now, what was the common theme there? Machine learning. In fact, he’s written and published 13 different books on machine learning and has been featured on Forbes, CNBC, Bloomberg, and more. Now, in his latest pursuit as founder and CEO of Plutoshift, he’s committed to bringing artificial intelligence to industrial organizations like manufacturing. Please welcome to the show, Prateek Joshi. Prateek, thanks so much for being here today.
[00:01:23] Prateek: Hey Josh, thank you for having me on the show.
[00:01:26] Josh: How’s today treating you so far?
[00:01:28] Prateek: Good so far. It’s a nice sunny day out here in California, so yes, it’s exciting.
[00:01:36] Josh: Well, that’s great. I’m here in Texas, and we’re in that weird time where it’s freezing in the morning, like literally freezing, and then a beautiful sunny afternoon. It’s about 72 degrees where I am, but it was super cold earlier. We start off each conversation by understanding what the day-to-day looks like for each of our guests. I’d love to hear from you Prateek, what’s a day-to-day look like in your role?
[00:02:03] Prateek: In my role, the ratio of how I spend my time, it might change, but most of my time is spent either with customers that is sales and customer success or hiring, how do we bring the best people? How do we get them to join Plutoshift or fundraising, basically making sure the financial future of the company is secure. That’s what I do for Plutoshift. On top of that, I love to keep myself abreast of the latest developments in machine learning. In my spare time, I write a newsletter. I’d spend time within machine learning communities, just making sure that I’m up to date. That’s how I spend my time.
[00:02:50] Josh: Machine learning has been a theme through your career, and as you just mentioned, when you’re not working, you’re still actively participating in the machine learning community, you’re sharing your own insights. You’re learning. It seems like machine learning is something you’re pretty passionate about?
[00:03:05] Prateek: Yes. It’s pretty much all I’ve done in my career. It started way back when I just was in school. It just made sense to me, and it has done a lot for me as I invested my time, and I got a lot back as well. It’s been a fantastic path personally.
[00:03:30] Josh: That’s great. It’s always great to find something that you’re genuinely interested in and then being able to make a career out of that. That’s how you don’t work a day in your life. That’s how that saying goes. Well, we’re talking today about artificial intelligence and machine learning, and we’ve actually talked about it on the show before on how artificial intelligence and machine learning is not BS, and that’s what [unintelligible 00:03:55] of Odin Technology shared with us.
Let’s be clear, it’s not BS. It’s actually pretty critical to the future of manufacturing operations. I would love it, Prateek, if you could take some time to level set with us on AI and starting with, in layman’s terms, what is artificial intelligence?
[00:04:15] Prateek: Artificial intelligence is the ability of a machine to do a task in an intelligent way. That’s something that requires intelligence to achieve the goal, and the way we relate AI, ML, and data is AI is the goal, and machine learning is a vehicle to get there, and data acts as a fuel for that vehicle. This is how, at least in my head, I connect these terms. You can infuse AI into any existing system. Meaning, there are systems that are just like, you add 10 numbers in a calculator.
You don’t need– it’s just computation, but as you infuse AI into any system, it just starts doing tasks in a more intelligent, more automated way so that you don’t need to explicitly provide a list of steps every time. It just learns. That’s how I think about AI being infused into systems.
[00:05:26] Josh: You started off the explanation with AI is the ability for a machine to do a task. I’m going to ask, probably, and the listeners are probably going to think this a dumb question here, but when we say machine, what are we actually talking about?
[00:05:40] Prateek: A machine could be a physical device, could be hardware, but also machine could be just software, meaning anything– again, I use the word machine in that sense something basically a system that can achieve a physical task or a soft task or anything in between as well. That, to me, is as a machine, and we see those examples appear everywhere. Meaning, if you infuse AI into a robot in a manufacturing plant, that’s a machine. You can infuse AI into your– for example, when you write your documents, you can infuse AI and detect spelling mistakes, suggest good sentences, correct the structure. There are many ways to infuse AI into machines.
[00:06:29] Josh: I love how you put it. AI is the goal, that’s the destination, and machine learning is the vehicle that lets you get there. All vehicles run on some sort of energy. The energy that it’s running on in this case is data. You do need data to feed the machine learning so that you can successfully have artificial intelligence. I feel like I’m learning so much already, but let’s break it down. We talked about what AI is. Talk to us about what it does.
[00:07:00] Prateek: When we look at how AI appears in our real lives, I look at it as a tool that augments whatever we do today. Again, ideally, the goal of AI is to just understand what needs to be done and do on its own, and we don’t allow to bother us. But today, the way it’s being used a lot is you have a certain task that a human is doing. They can continue to do it, but by infusing AI, they can do it a whole lot faster with zero errors, and that level of augmentation is where we are finding a lot of success, and those case studies appear everywhere.
A simple analogy would be, if you want to go from here, like California, or San Francisco, to New York, you can walk. That’s one way to do it. It’s just going to take a very long time. You can drive. That’s like infusing a little bit of AI. You can take a flight. That’s more advanced AI. Basically, going from point A to point B faster, better, cheaper by augmenting yourself, that’s how AI appears in real life.
[00:08:14] Josh: I like how you use the term augmenting, whereas I think a lot of times when people think about AI, it gets looped in with automation. Can you talk to us about your perspective on artificial intelligence, that idea of augmenting versus automating?
[00:08:33] Prateek: Automation is– a simple example would be, you’re doing a task, and let’s say it has seven steps. Step one, step two, step seven, the work is done. Automation is literally defining what those steps are with absolutely zero room for error or variation. Let’s say you build an automation system to– somebody inputs a receipt, you extract the information, you do this, and then you file it into your accounting system, and then you’ll do the report. Great, fixed system.
Now, if for some reason, let’s say somebody forgot to put in a number or the number scratched out or something happens, the whole thing just stops, because guess what? You slightly deviated from the tool. The automation tool that you have built is just full stop. Now, AI is a layer above that. Meaning, automation, yes, sure. To automate, you can infuse AI into an automation system, but AI is not just automation.
One way to think about it is now you take the same system, the receipt filing system, and you can infuse AI that can detect, “Hey, that number is missing, maybe paying mark,” or, “Hey, in step three, I encountered this anomaly. Maybe paying Angie and ask here what’s happening.” It’s basically using a system to infuse intelligence. Yes, automation is one form, one way of one application, I would say, of AI, but in no way is it limited to automation.
[00:10:10] Josh: Back to the idea that you brought up of augmenting, and the examples you used to illustrate, you can walk to San Francisco, you can drive to San Francisco, you could fly to San Francisco. The central theme there is augmenting the person. It sounds like from your perspective, artificial intelligence is not a replacement for people or for human decision-making. [crosstalk]
[00:10:33] Prateek: That is correct. That is my viewpoint and also a lot of how I use AI in my own life. Again, it’s just a tool built by others. The way I use it, a simple example is AutoComplete. I can technically sit and type everything out, but AutoComplete is getting good when I write Gmail-like emails, I write word documents. It helps. The simple sentences, it autocompletes, I’d have it, and great, it works.
It’s like little, little things that just work. That’s one example when I am searching, like when I go to Google when I search for something. I can technically look at all the web pages and pick the correct one, but somehow, they surface the right one, I click on it, problem solved. It’s like all these little things that we use every day, it’s already augmenting our work. That’s how I think ML and AI can drive success and become part of our daily life.
[00:11:35] Josh: It’s really about when we say augmenting, we’re saving time because I think the point that you’re illustrating is you can do these things without AI. It’s just going to take a long time and dedication and effort, and I think we’d all agree that time is– it’s difficult to prioritize anything but the immediate production needs of the day, especially in manufacturing where the work the workforce is really complicated right now.
Getting people into be those people who figure out who– you need to augment the people that you have because there’s a lot of people that you don’t have who would be critical to spending the time to do that. Back to your example of AI being the goal and machine learning being the vehicle and data being the fuel, that raises a question to me this idea of what is actually needed to get started with artificial intelligence going from nothing to actually bringing and infusing your work with artificial intelligence.
[00:12:42] Prateek: It starts with identifying the work. Meaning, well, firstly, the work or the human– let’s say there’s a team or a team of people who are already doing a work or a workflow it’s already important. It’s part of their life. That’s a great starting point to bring in AI because a lot of times, what happens is people have AI tools and then look around, “Okay, what can I use it for?” That’s just a completely reverse way of looking at it. Look at the work you’re already doing today. Then, within that, break it down into sub-parts a little bit, not too many parts but conceptual parts.
Then, look at what’s being repeated over and over again in very– steps that almost never change, something that lends itself nicely to automation is a great starting point. It’s low-hanging fruit, something that can be automated with high accuracy. When you do that with AI, it builds trust. What are the ones you’ve identified and then the implementation part is where you collect the data. You clean it, you build the model, and you infuse it into your workflow so that steps one to step seven, within that, maybe humans are only needed from step four to seven. Steps one, two, and three can be done by maybe an AI system that can just finish a task for you. That’s how you bring in AI. It’s basically very– you start with that. It’s not a top-down approach It’s more of a bottom-up approach here.
[00:14:32] Josh: Yes, so it’s not AI for AI’s sake. It’s how can AI augment a particular aspect of a well-defined repeatable process? Now, you mentioned something specifically. You said, after you’ve identified the process, and you’re getting started within the implementation, you have to collect the data, and then you build the model. As you mentioned before, data is the fuel for the vehicle. That aspect of collecting data, what type of data needs to be collected, or what data do you need to have to start with in order to build these models that then lead to AI?
[00:15:09] Prateek: The type of data that you need depends on the task at hand, basically what model you’re building. A simple example is, does this car need gas? Is it an electric car? Does it need diesel? Like what does it need? In this case, the type of data usually relates to the goal you want to achieve. A simple example is, let’s say you want to reduce electricity consumption at your facility. I know you’re a manufacturer, you identify the goal where I got to reduce my electricity consumption.
The data has to relate to that. Meaning, historically, let’s say you look at the last 12 months over the last 24 months, and you gather data related to electricity consumption, and also, what metrics or what levers can you pull to impact that. A simple example, pressure, temperature, and flow rate, is basically the metrics that you can move around to impact electricity consumption. Once you have the data of the target and the data streams that impact that, then you get started on building the model basically to achieve the task.
Now, when you look at the model, broadly, you just need to know what direction are we heading? Meaning, are we building a system that needs to give out a yes-no type of answer, or are we building a system that needs to predict a number. Or are we building a system that needs to tell me what’s going to happen 48 hours from now? Based on these broad directions, you set out and you build the model, and then it becomes part of it. That’s how we look at bringing it into the system.
[00:16:58] Josh: It is very much in line with that idea of starting with the process and finding those things that need to be augmented. Then, I love that you clarified that the data really depends on the goal, especially like your example, is it supposed to predict something? Is it supposed to provide a recommendation? Is it supposed to just report out on something? Each of those impacts the types of data that you’re probably going to need.
In my experience, there’s a lot of data points that aren’t readily available. Do you find that you have to spend a certain amount of time just guiding people on, “Here’s the data that you need to gather over this period of time?” If so, are there any struggles that you typically see or any obstacles that the people need to be aware of?
[00:17:50] Prateek: Yes, it actually happens a lot in the real world because when you look at the data that you need, more often than not, you have some data and maybe there are some missing points. The goal is, step one, after you identify that, “Okay. I will now reduce electricity consumption. I need these 11 data streams.” Within that, let’s say you realize that “Oh, I never collected flowrate data or temperature data.”
The goal is, once you know what you have and what’s missing, you can set out and start collecting the data. That’s one part of it. The other part is, and in the last probably a decade, machine learning systems have become great at training on a lot of data. Meaning, you collect insane amount data, and yes, sure, the model will be good because there’s so much data, but in the real world, especially in the physical world, like manufacturing, the level of data, the amount of data is not big.
It’s not big data because, for example, the number of sentences you have available on the Internet, it’s insane as compared to the sensor data you need to predict an outcome in manufacturing. There’s a whole new school of thought coming around small data. It’s actually very nice– a lot of leading machine learning researchers and practitioners are advocating how do we get machine learning models to train on small data when the amount of data is both limited by time, and also it’s sparse?
This means, for each thing, you don’t have a trillion parameters. Now, you’ll have a very limited set of data. Now, how do we build the models to account for it and then build systems? Because in the real world, you won’t have a huge, huge amount of data that typically an image recognition system has access to.
[music]
[00:19:55] Josh: Hey, we’re going to take a real quick break to hear from our sponsors. Stay tuned for more. Conquering Chaos.
[00:20:02] Rob: Hey listeners, it’s Rob. I’m one of the producers on Conquering Chaos. I’m right here with you for every episode, working behind the scenes to make sure everything is just right for your listening experience. Whether you’re a new listener, binging content to help you conquer the everyday chaos or a dedicated fan tuning in for each new episode, there’s one thing to always keep in mind. Information is useless unless you use it. Obvious, right?
It’s so easy to learn, forget, and then miss out on the opportunity to make real improvements to day-to-day activities. Well, the folks at Parsable have an opportunity for you to learn, experience, and make real improvements to those same day-to-day activities. Get rid of paper on the factory floor. It’s the quickest and easiest way to make a measurable impact on safety, quality, and production. Think about it.
Paper-based checklists, forms, and SOPs isolate workers from getting the information they need when they need it, which leads to a number of inherent inefficiencies that you probably accept as a part of your own everyday chaos. As a result, you can’t respond quickly to problems, you struggle to standardize the completion of critical tasks, and you miss out on new continuous improvement opportunities.
Parsable is proven to help across a number of different functions, including autonomous maintenance, line changeovers, in-process quality checks, and more, which has helped industry-leading manufacturers reduce unplanned downtime, increase OEE, improve throughput, and more. See for yourself how easy it is to bring a connected digital experience to your frontline workers by using Parsable risk-free for 30 days. Check the show notes for the link. All right, back to the show.
[music]
[00:22:05] Josh: That’s an interesting point. I’ve certainly heard of big data. I never heard the term small data, but when you say that, it immediately makes sense. I’m imagining something’s a little more focused and understanding of the fact that you do have less pieces of evidence to work with. The model needs to be adjusted to work that way, to work with that constraint. Well, let’s talk about artificial intelligence in today’s manufacturing problems.
One thing we should all keep in mind is that, and you brought this up a little bit earlier, artificial intelligence is already an active part of our lives. When you Google a topic like you mentioned the autocomplete of the search results, when your phone– I don’t know if everyone listening knows this, but if you have an iPhone, you can go to your photos, and you can type in a keyword, it could be a person’s name.
Whenever I want to pull up pictures of my dog, I just type in dog, and it brings up every picture on my phone with a dog in it. When you’re streaming music station, plays the exact song that you want to hear, or sometimes the complete opposite, that’s artificial intelligence working in the background for us as consumers. It’s helping us solve problems that we didn’t know that we had or problems that we do know that we have, and it’s saving us time.
It’s saving me time of finding my dog’s pictures. It’s saving me the time of what song do I want to listen to next. This is something that’s all around us. Prateek, I’d love to drive the conversation towards how artificial intelligence can help manufacturers today.
[00:23:44] Prateek: Today, the main or the most important use cases are centered around resource consumption or increasing the throughput, basically anything that directly impacts either the top line or the bottom line. There’s a lot of success being driven on that front. If you go a level deeper, and it comes to resource consumption, resources, such as electricity or chemicals or water, these are very important resources, and also, they’re very expensive resources to a manufacturer.
If you are a manufacturer and you are using these resources to make your product, could be ketchup or cheese or any number of products, the goal is to understand how do we reduce the resource consumed per unit of output? That means there is many things you can do. Either you can– Simple example, let’s say you’re a beer company, or let’s say you’re a food processing company, and you use a couple of millions of gallons of water per day, and you use dozens and dozens of membranes to clean the water.
Now, as the membranes get blocky, you clean more water, they get blocky, and then, the efficiency goes down with time. Meaning, you’ve got to push the water harder and harder to get it to the other side. Now, that’s the normal way of operating. Now, with AI in the mix, it will identify that, “Hey, this membrane is so blocky that you’re spending more on electricity to push the water through as opposed to just running a quick acid wash. Wash it and then run the system again.”
Washing is a cost. To clean a membrane it’s expensive. Basically, what it does is, instead of spending weeks and months just paying more and more electricity bills, it’ll tell you that membrane number 79 needs to be cleaned today so that it stops consuming five x the amount of electricity. This is a simple example of how AI can be used to solve a specific problem, in this case, electricity consumption, and bring it to life. Again, this relates to the earlier conversation about identifying a piece of work that’s already being done, in this case, cleaning the water. With or without AI, they’ll do it. With AI, they can do it better, faster, cheaper.
[00:26:25] Josh: Another thing you mentioned, you said resource consumption and throughput, I think that was a great example of resource consumption. Talk to us a little bit on how it can help on the throughput side.
[00:26:37] Prateek: Yes. With throughput, the goal is to track the metrics that impact the throughput. In this case, let’s say– actually, I’ll take the same example and flip it a little bit. Now, let’s say that you already have the budget to spend, “Hey, I’m going to spend this amount on buying electricity.” Great. I don’t want to change that, I don’t want to save electricity, which means, can I produce more units of food or beverage or chemicals per unit of resource consumed?
That way, now, the problem shifts from that to tuning some other parameters. I’m going to keep this constant, and how do I identify and [unintelligible 00:27:17] the right levers that I can pull today to increase the throughput? Again, it’s the same example, and the reason I’m using the same example is just to demonstrate how fluid and how it can appear within your process without ripping the whole thing out. That’s another example.
[00:27:38] Josh: I think you’re bringing up a good point on. Well, this is a little bit tied to the previous point that you made is the data points that are needed for some of these initiatives, and some of them you may have, and some of them you may not have, and this is a conversation that we have pretty frequently here at Parsable. We believe that there is quite the impact that frontline workers have on manufacturing operations. Let’s take the example of throughput.
One of the most common situations that we see is there’s a struggle to ensure that the autonomous maintenance activities that need to get done are actually getting done, and if they are getting done, are they getting done the way that they’re supposed to get done? I bring this up because when we’re coming down to data, at that point, we’re coming down to, can you get data on the activities that people are doing or aren’t doing, and how does that tie to your other operational data points? For example, if there’s a problem with throughput, how are these variables impacting that conversation?
When you layer something like artificial intelligence on top of that, now you’re getting all the data points on these different variables, and you can walk away with some correlation or some understanding of, “This is what seems to be the case based off all the data and the model that’s running. Here is the action that we can take to ultimately impact something like throughput.”
[00:29:06] Prateek: That’s actually a great, great way of looking at it. Actually, I believe a better way to bring AI into the mix today is use it to detect events of interest. Prediction is great. Forecasting is great, but detecting an event that’s already happening and bringing it or surfacing it is not one– it has an immediate impact. People will be able to take an action right now. Two, it builds trust. Meaning, let’s say, if I predict, “Hey, this is going to happen three months from now,” people, “Well, not sure,” whatever, like yes, who knows? Three months is too far. If I tell them like, “”Right now, membrane number 75 is eating up five x more electricity, you can go check.”
That is like an instant win. They’re like, “Oh, thank God. I didn’t have to check 300 membranes. I know where to go, and I fixed it and has an immediate impact on it. Detecting events of interest and even within that, surfacing the most important one at the right time, that’s a great way of bringing AI. Then, once there’s trust, once it learns how to work with the system, then yes, you can predict events, 48 hours out. You can forecast. You can do all the good stuff.
[00:30:21] Josh: When you brought up resource consumption, throughput as examples, we’re breaking those down. There’s elements of inefficiencies that need to be addressed that you may not be aware of that can be surfaced with the data. There’s elements of tackling ESG initiatives. Like you said, if you’re lowering the overall total need for energy consumption or even the example that you provided, if you’re able to– now, you don’t have to do a specific cleaning, and that saves on let’s say water consumption or the use of chemicals, for example.
That’s one of the ways in which it ties into your ESG goals. Is there anything surrounding retention, recruiting knowledge share that in your experience artificial intelligence can help with?
[00:31:11] Prateek: Yes. Couple of things here, then, one, as you said, by doing this, by reducing energy consumption, chemical consumption, water consumption, that’s a direct impact on your ESG goals for the year. Also, it puts the company in a great position. So, as we enter 2022, ESG is becoming– it’s a very important topic. It’s entering people’s consciousness a lot. We talk about it, and also, when it comes to– When you’re looking to bring on great people, it matters that, as a company, you are doing something beyond the revenue, beyond what you are required to do anyway.
I think on multiple levels, just as a good company, as a good steward of capital, as a company that contributes to the environment, and also as a company that can attract the best talent, because you’re taking that extra step, you are making an impact. I feel like some of the world’s best professionals, they care about it. They want to do it, and obviously, it doesn’t mean you have to do voluntary work. It’s not– It’s a business, it’s still a business, but the goal is becoming a differentiator. It’s good for the environment, and it’s good for the company, too. I think ESG, we are seeing a lot happening now, and I think the momentum will continue.
[00:32:42] Josh: I certainly think so as well. If we sum up some of what we’ve talked about, it seems like a fair assumption or a fair conclusion, I should say, is that artificial intelligence, it seems like it’s more for people than it is necessarily for systems.
[00:33:01] Prateek: Yes, I would characterize it that way. It’s for people.
[00:33:06] Josh: It’s for people. What would a mature implementation of artificial intelligence look like?
[00:33:12] Prateek: A mature implementation is when you don’t even know it’s there. That’s when you know it has come to a point where you don’t even realize it, it’s just there. It’s doing its work, and you think that, “Oh, it’s like me doing the task,” without even realizing you are being augmented by an AI system.
[00:33:33] Josh: Got it. It’s not necessarily in the background, but it’s such a cohesive part of the day-to-day that if it were gone, you would notice, but as long as it’s working, you don’t necessarily realize.
[00:33:47] Prateek: Yes. That too. That, to me, is a success. I’ve seen that happen a lot with over the last decade. Good technologies, they start with this and that was separate and then they become part of it, but you can still see it. Then, it’s so ubiquitous that you barely notice it anymore. Then, that’s how I like it anyway.
[00:34:10] Josh: Let’s shift gears a little bit. You’ve written the book on artificial intelligence. Like we said, in the intro, your background is in machine learning, and now, you’re leading a company that provides a data platform for automated performance monitoring and predictive analysis. You went from tech to serving in industrial organizations. Talk to us how you found your way going from tech into industrial organizations, like manufacturing.
[00:34:39] Prateek: When I started in machine learning, I spent a lot of time building products in the virtual world. It could be images, text, videos, anything that appears online and obviously explored a variety of domains and subdomains and realized that through this process, AI is ubiquitous in certain fields, like search engines and e-commerce, but when it comes to the physical world, there’s a pretty big gap. It’s not nearly as ubiquitous, and that was the core motivation behind launching Plutoshift is to bring AI to the physical world and bring the maturity to a point where you don’t even know it’s there.
It’s just doing its job. The task is– the work is being done. The human is being augmented with this AI system in the background. That, to me, is a very exciting field because when it comes to the physical world, it’s a new frontier for AI. When you look at, say, a marketing tool or a search engine, it’s been done. People have implemented a thousand systems available, but physical world represents a new frontier, and there’s a lot of opportunity for AI to make an impact. That was the core motivation to doing this.
[00:36:06] Josh: I can certainly relate to that perspective. Sometimes I spend all my time in the digital world, creating something digitally, like a– a classic example is a PowerPoint. I’ve spent so much time on PowerPoints, and I’ll leave, I’ll end the day, and I’ll think, “What did I actually do? What do I have to show for it? There’s nothing physical.” If I can’t access the Internet, I might not be able to access this thing I spent so much time working on.
That’s certainly something that I love about manufacturing is, at the end of the day, you’re putting something together that’s physical that consumers rely on, that people rely on. I can certainly resonate with your transition into serving the physical world. Talk to us a little bit about Plutoshift. What problem are you solving for manufacturers and how?
[00:36:55] Prateek: Plutoshift is a data platform for an automated monitoring. Meaning, as a company, let’s say you are a beverage company, and you have physical infrastructure. Basically, raw material resources they go in, and product comes out. Within this infra, the goal is to keeping an eye on your infra is just part of their daily lives. If you’re part of the ops team, you have to keep an eye on ideally every component of your infra, and hopefully, you’ll know if something is going bad. Now, in this modern world, 2022, it’s rarely– there’s no catastrophic– systems don’t go down every day.
What’s way more frequent is a system starts being inefficient. Meaning, instead of consuming electricity at a certain rate, it starts consuming 5x. It’s still functional. It still keeps going, but it just sucks up a lot more of your resources. So, identifying– and sometimes if you just do it manually, it’ll take weeks or months before you know that, “Oh, that part of the system has been using up 5x more electricity.”
That is where a monitoring platform comes into play. It keeps an eye on all the different components of your infra, membranes, cooling tower, heating systems, boilers, condensers, clarifiers, any asset or process that appears in the physical realm, so a good monitoring platform keeps an eye on that. Then, if something happens, something an event of interest, it detects it, surfaces it with the right time, and then allows the operator to take action.
Also, it predicts, “Hey, if you don’t do anything in 72 hours, this is what’s coming next.” Detection and prediction go hand in hand when it comes to manufacturing. That’s what we do. We serve Fortune 500 customers who use our platform to monitor their infra.
[00:39:05] Josh: It certainly sounds like it’s living up to the principles that you described of augmenting the human, making it easier to find out that a problem’s occurred so that you can take action before any devastating downtime makes its way into the fold. Talk to us a little bit about what makes Plutoshift stand out.
[00:39:27] Prateek: I think it’s the core understanding of how to productize this offering. Basically, what happens is, usually, when you go to a manufacturing company, they have two large buckets in terms of options. Meaning, if they want to keep an eye on their infra, they can either do it– actually, the status quo is asking the operations team to do it manually. That’s the status quo, and if they want to do something new, either they can use Tableau or Power BI. Basically, it’s a tool, like Excel. You put in the numbers, and you can create graphs and charts, but it’s just too generic. Meaning, the same tool is applied in healthcare and e-commerce and also manufacturing. It’s not built for manufacturing. There’s a lot of work to be done by the ops team to make it useful. The second bucket in terms of options is you can hire or you hire a contractor, like a large consulting company or contractor, and they can build a very customized system for you.
It’s going to take five years and many millions in budget. There’s a big gap in between very free, inexpensive tools, or a very, super expensive consulting firm. There’s a big gap to productize this offering. That’s why we were able to productize this and deploy it only for the manufacturing field, so it allowed us to stand out and also make sure it’s impactful and useful for our customers.
[00:41:04] Josh: In my experience, because we certainly run into Tableau, Power BI, build your own consulting firm come in, a variety of different solutions like you’ve talked about, that idea of building it yourself, like setting up Tableau or Power BI, I’ve certainly seen some of the difficulties be– sometimes these tools can be pretty complex and require a background that not everyone currently within the operation is able to support. Can you talk to us a little bit about who can use Plutoshift? What sort of technical background or skills would they need to have?
[00:41:43] Prateek: The Plutoshift platform is built for the operations teams, and the typical user profiles include an operations manager, operator, regional manager, director of ops, VP of operations. Basically, VP of ops and everybody who reports to this person can use the product. It’s specifically built for the operations team, which means that you don’t need to know data science or AI or anything.
Basically, the goal is somebody who knows physical infra and somebody who knows what a metric would mean, that’s pretty much it. It’s built for them. They can drag and drop, they can customize it, make it their own, but they don’t need to know anything about databases or data science, AI, or machine learning.
[00:42:34] Josh: That’s good. That’s certainly important. Sometimes we talk about technology that would be great and revolutionary but requires a skill set that don’t necessarily have at the time and you got to balance, how do you up-level the talent that you need to take it to a point, but also, are you choosing the right solutions for you, and the team that you have current day? I love digging into that topic.
Well, Prateek, we’ve covered a lot. We covered what is artificial intelligence, what makes artificial intelligence run, how to get started with it, how it can help manufacturers, how Plutoshift came to be, and what some of the value points that it brings forth. I’d love for you to share how listeners could continue the conversation with you or learn more about Plutoshift.
[00:43:23] Prateek: To learn more about Plutoshift, you can visit plutoshift.com. We have a lot of good information there. If you want to get in touch with me, feel free to contact me via LinkedIn. Just look me up, Prateek Joshi. Also, if you want to follow my newsletter prateekjoshi.substack.com, where I discuss machine learning concepts, how do you build products. I think, yes, I’m happy to continue the conversation.
[00:43:52] Josh: Well, great. Well, we sure enjoyed having you. Thanks for joining us, Prateek. We’ll certainly be on the lookout for opportunities to augment people with artificial intelligence.
[00:44:04] Prateek: That’s great. Thanks, Josh, for having me. Really great conversation.
[music]
[00:44:11] Josh: That’s the show. Thank you so, so much for joining us today. Conquering Chaos is brought to you by Parsable. If you’re a fan of these conversations, subscribe to the show and leave us a rating on Apple Podcasts. Just tap the number of stars you think the show deserves. As always, feel free to share what’s top of mind for you and who you think we should talk to you next. Until then, talk soon. Take care, stay safe, and bye-bye.
Listen to find out how Machine Learning & AI improve manufacturing