Why Manufacturing Needs People Analytics
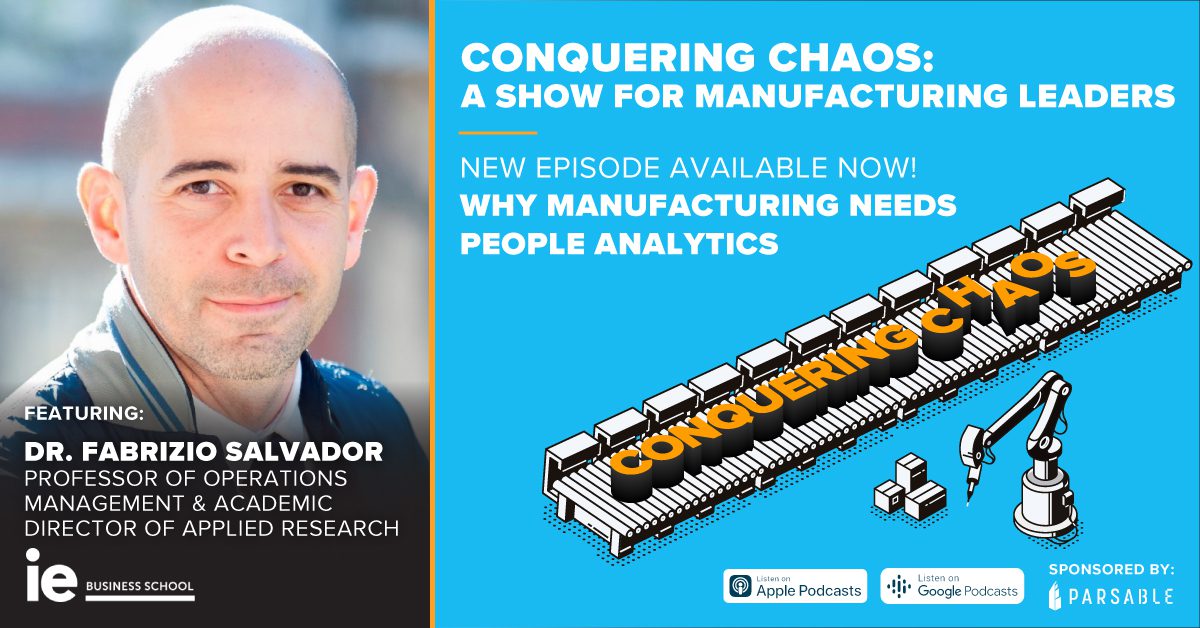
In this increasingly connected world, data plays an integral role in understanding and improving businesses. People analytics, in particular, uses data to improve how businesses leverage the creativity and talents of their people.
In this episode, Dr. Fabrizio Salvador, Professor of Operations Management – IE Business School, shares how people analytics helps organizations deploy their personnel more effectively and ensures they are doing more of what they do best. What manufacturers can learn from people analytics may surprise you.
Check out the resources below for more information:
Visit www.parsable.com/podcast to join our mailing list.
Use Parsable risk-free for 30 days .
Take a look at our Demo to learn more about Parsable.
We discuss:
What people analytics is
What people get wrong about it
How to operationalize it
______________________________________________________________________________________________________________
Are you ready to start your digital transformation journey? Request a demo today.

______________________________________________________________________________________________________________
Check out the full episode below:
Fabrizio: People analytics is not only NHR responsibility is every unit’s-
[music]
[00:00:14] Josh Santo: Welcome to Conquering Chaos, the show for manufacturing leaders. In each episode we are connecting you to the manufacturing leaders of today who are driving the innovations needed to Futureproof the operations of tomorrow. If you feel your time is spent fighting fires and trying to control the everyday chaos, this show is the show for you. My name is Josh Santo. I’ll be your host.
Hey all, it’s Josh. Before we get into this episode, I wanted to put this into your ear. If you like the types of conversations we’re having, you’ll enjoy the content that we share through our mailing list. Go to parsable.com/podcast, scroll to the bottom of the page and sign up to get more insightful content delivered directly to your inbox. On to the show.
Welcome to the show. In today’s episode, we are going to be more than listeners, we’re going to be students. Our next guest is an operations and technology professor currently working at IE business school which is one of Europe’s highest ranking business schools, and also spent I as an educator at other well known universities like MIT and Arizona State University.
In fact, Thinkers 50, which is a leading institution focused on identifying and sharing new innovative and compelling management ideas selected him as one of the top 30 thinkers to watch in their radar class of 2019 and described him as an outstanding conceptual thinker and passionate champion of operations. Passionate is the key term here. What he is passionate about is using analytics to understand how organizations can better deploy and recombine their personnel into their core processes. Please welcome our next guest, Dr. Fabrizio Salvador.
[00:02:11] Dr. Fabrizio Salvador: Thank you, Josh. Thank you for the invitation. Thank you everyone for listening this podcast. Great to be here. Ready for an interesting conversation with you.
[00:02:23] Josh: That’s right. We’re really looking forward to picking your brain and ultimately serving as students here. Now, the way that we like to kick off each episode is just hearing a little bit more about our guests particularly what their day to day looks like. I’d love to hear from you, what’s a day look like in your role?
[00:02:42] Fabrizio: It’s an interesting question because my day reflects the fact that I wear multiple hats professionally as you correctly said, I’m a professor of operations technology so I need to do research and teach and these things, but also I am a vice director for applied research which is more of an administrative assignment within my university even though that entails let’s say, concept of work too.
I have my own practice of training and consulting. How I juggle all these different professional responsibilities, well, I apply the principle of sparing my best time for the most difficult part of job and then using the rest of the time for others. Since I’m a morning bird, I try to block the first few hours of the morning for research thinking. I try to avoid meeting if possible and then I have the meetings and class maybe in the afternoon and if I’m unlucky, in the night. That’s a little bit the ideal state. Then perfection is telling me of good. Sometimes your schedule doesn’t exactly reflect your wishes but I try to be disciplined.
[00:04:05] Josh: That’s something that comes up pretty frequently on the show. There’s usually some routine that the leaders that we get to speak to have. Very focused on the morning routine actually. It sounds like you’ve got a lot on your plate. You have to be as disciplined as you can be so that you have those times to pursue what you’re looking to do. Now, I’m curious, Fabrizio, how many classes are you teaching a day?
[00:04:34] Fabrizio: Oh well, I think I will disappoint you because my teaching load in a year is if you translate into hours of or by the class, it’s something like 105 hours. It’s not really much. I take a little bit more because sometimes I get interesting teaching assignment that let’s say convince me to go under my baseline. I think that too teaching well, you can do too much of it.
[00:05:09] Josh: I think teaching is a very difficult and draining skill. I don’t mean that in a negative way. I mean that the person up there who has the information to convey to a diverse group of students, there is a lot of focus on that individual professor to adapt their message to the different learning styles in the room. It’s quite a difficult task. I can completely understand that even though it may not be a tone of classes, it’s still a ton of work that goes into it.
[00:05:46] Fabrizio: A lot of energy. I find teaching rewarding. Sometimes when I talk to colleagues that say that research is good, teaching yak. I think that teaching is one important way for us to give back to society at different levels. Essentially, you may think that I’m naïve but I think that teaching is an act of love because you are trying to do the best that you have. You do that with a loving attitude but that doesn’t mean that it’s not work. Even more. It takes really a lot of energy. I would say that teaching one hour is working three.
[00:06:30] Josh: [crosstalk] is super underrated and such a critical part of society. Responsible for helping young minds or really minds of any age, doesn’t have to be young minds, understand, discover and then that sets up society for whatever those next innovations are going to be or those next breakthroughs or those next policies. I think it’s incredibly important. A lot of respect to the educators out there and a lot of respect to you as well for being a professor.
Today we are talking about People Analytics. Now, it should be no surprise that in conversations or shows of content about industry is going to some way bring up data. In fact, it seems most conversations we have is about data, just data, data, data. It would almost be an understatement to say that everything is about data both in our professional and personal lives. When we think about this increasingly connected world, there are many different systems that we end up working with or that we end up using and interacting with and that we start to rely on. These digital systems they put out what some people refer to as digital exhaust. Which is an almost limitless supply of data points.
Then the question becomes, how do you use the data points to really discover insights, to take action, to make improvements, to really understand what’s happening within your business? With this data organizations buying the insights, and we’re talking about today how the right data from different teams, from different people used in the right way can make an impact. Tell us a little bit about what people analytics is and how it manifests for organizations.
[00:08:30] Fabrizio: The fundamental idea of people analytics is that human capital is probably the most important asset for the majority of organizations. Of course some organization high tech have a lot of patents and they may have some unique resources but I would say that across the board, people make a lot of the difference. Technology can buy data off the shelf, it can imitate it– imitating let’s say a social system within organization is very difficult.
The fundamental idea of people analytics is really how can you use analytics to get more from these people? Not in the sense of of squeezing them or monitoring them but instead of helping them fulfill better what human beings are good at doing. Thinking out of the box, connecting, elaborating, applying creativity rather than executing over and over a tasks.
[00:09:35] Josh: I that’s those are interesting points. I think that’s a good high level definition. Really the core of what you’re saying is that people make the difference. It’s not technology. In some cases, conversations come up about how tech and automation might be replacing jobs. In certain situations, that is going to happen. The argument here is not that people analytics is how you can force people to do more than what they’re capable of doing.
It sounds like people, analytics is about getting an understanding so that you can empower and enable the people that are ultimately bringing the value to your organization. This is a key concept of lean. In fact, there’s the seven ways, they also introduced the eighth way, which is, are you leveraging the creativity, the talents of the people that make up your operations?
It sounds like people analytics, these concepts that we come across in manufacturing of how do you empower people and really make sure that they are able to bring those human talents, which, like you said, thinking outside the box, creative thinking collaboration, how do you use that? How do you use data to identify that, empower that, foster that in drive innovation through the organization?
[00:10:55] Fabrizio: Absolutely. I really love the fact that you bring in this idea of the eight type of waste. The waste of human creativity and talent. I think this is something that organizations are really getting to understand that very well. As technology progresses and develops, we see not only automation of manual work as already happen a lot in manufacturing for the last a hundred years maybe, but also we see automation of clerical work and once was only automation of like accounting or payroll, now we’ve robotic process automation, intelligent process automation and all this technology.
A lot of the non-creative but sometime complex activities that usually people have been doing are being taken by machines. I would argue that this is good news. Of course, on the short run can create problems that we cannot ignore. I like that Wiener, the father of cybernetics used to say that every time I see a human being do work that a machine could do, I feel pity for the person. I think with a sense of compassion, we should really think about this. People should be doing something that had them fulfilling their work.
[00:12:27] Josh: The core of what you’re bringing up is the intention is not to replace people. The intention is to take off certain responsibilities off their plate because there was no other options. How do you free up that capacity of the individual to contribute to higher value producing activities? That leads me to one of my next questions, because I could imagine that when people first hear about people analytics, or even in common implementation of people analytics, people aren’t getting it a hundred percent correct. I would love to hear from you what your perspective is on what most people get wrong about people analytics.
[00:13:12] Fabrizio: Okay, this is a good question. Rather than what people get wrong, I think that people get a too narrow view of what is people analytics. In industry, when you, let’s say, recall this labor thing, people analytics, automatically people are, okay, the guy is talking about HR and analytics. If we think about the typical steps of the employee journey as handled by the HR unit, like selection, recruiting, onboarding, development, performance evaluation, upskilling, and eventually leadership or exit, that’s great.
All these activities can be supported by people a analytics applications that actually pretty well-known, even though the progress is small, but there is another side of people analytics that at least as important and the organization struggle to see. What is this about? Well, if we think that it’s true, that that is a life cycle of the employee from the HR perspective, but normally employees within organization work in verticals, in business lines, in the divisions, in different units, factory plants, whatever kind of organizational structure that are linked to product market combinations.
The real point there is, how do algorithms and statistical inference can help me in the day today management of these employees? You see that this goes beyond what is normally the right use of action of human resources. Because goes into the vertical common chain of the division or the unit. A lot of decisions are there. Let me give you a couple of examples here. When we talk about software maintenance organization, typically, these organization handle thousands of request per year. What they call tickets. Every time a ticket gets into a system, you have a question, who do I assign the ticket?
In most organization, this assignment is done by a manager who is extremely under pressure, doesn’t really have time to think who’s the best person to handle this. He just need to get the thing out of the queue, or in other more complicated project context, the question becomes, Hey, you have to put together a team to make a bid for a certain opportunity, a commercial opportunity, who do I put into the team?
Again, same problem. We ask managers oftentimes to do this type of routine decision that depends on many variables at the same time. Our homo sapiens mind is not good at handling many variables at the same time, many times per day or per week repetitively. The question therefore, you see that this people analytics becomes a responsibility of the business lines.
Of course, HR can have a say here because it can be as Peter Cappelli from Wharton says, it can be a supporting actor helping the business unit with crossing their data that they have about people with the business unit, data on the people and create application, but this is what we often miss. The fact that people analytics is not only an HR responsibility is every unit’s responsibility.
[00:17:17] Josh: I think that that’s a very compelling description of how people analytics is boxed in to this idea that it is HR, but really the entire organization, particularly for thinking about frontline operations. The more you can empower people with the data to make the best decisions in the moment, there’s going to be a big impact. Part of the consideration is the people factor. People analytics can really help augment decision-making by these frontline supervisors and frontline managers, for example.
I think you mentioned this, which is minds are not good at handling variables. I completely agree. A lot of the work that we do focused around process improvement and augmenting people with technology. You can almost go down a black hole of variables. You’ve got who’s on shift, what’s their experience, what’s their background, what’s their state of mind at that time?
You’ve also got your variables not related to the people which could even include what’s the temperature of the weather? There’s so many different factors that come into play that the mind can’t take in everything at once. When we’re talking about how do you make the best decisions? You have to have data to really understand that and drive at it. Especially when we start talking about more advanced technologies like artificial intelligence and how that can help sift quickly through variables and different sources of data so that you make these best decisions.
What I love about the example that you’re providing is that it’s not just about information that HR needs, it’s how can you equip the people who need to make these decisions in the moment with another critical factor about leading an organization, which is your people. I think you gave some good examples of how we could recognize that in our day to day, but I think it would be interesting to talk about the impact because we’re talking about what it is, let’s talk about the impact.
When you look at manufacturing in particular, and this is not just isolated to manufacturing, a lot of industries are experiencing this, people are the priority right now. There is a struggle to bring in new people and to retain talent. It sounds like people analytics could play a part in helping there. Not necessarily– Certainly in the recruiting part. I don’t mean to say that it couldn’t help that, but what I mean is the retaining part, the empowering part, the upskilling part, which are all critical components of having a successful and satisfied workforce. I’d love to hear from you, in your experience, what are organizations able to accomplish by leveraging people analytics in this way, in a way that’s not just focused on HR?
[00:20:22] Fabrizio: It is a broad question. I will go by examples first and show you different things that can be achieved, and then we will probably generalize, so I will rely on your further questions. What can an organization achieve? Let’s jump into the global construction industry. A client that I have is one of the global leaders in the cladding of skyscraper, super tall towers and large public buildings such as airports.
They have a truly global network of offices, design facilities, engineering facilities, manufacturing and fabrication plant, and then they have the sites where they actually dress up a building. I’m talking about like the Apple headquarters in Cupertino was one of their projects, but there are hundreds of these. What happens here? The company originally, like many company, had been growing by means of mergers and acquisitions. They had what we call a confederated structure.
What happened is that every time an architect like Zaha Hadid, when she was alive, or Renzo Piano or whatever kind of an architect you like, came to them and specified one of these freaky buildings, the tendency of the project technical managers was to play safe and not use too many units even though they were apparently very skilled in some aspect of the project for control purposes. We tried to solve this by means of discussion and you never go anywhere there.
I said, “Let’s take a business analytic approach applied to people.” What we discovered, we codified data about something like 200 projects. We codified data about who was the technical leader of the project and how much they were really relying on distributing the project across different offices because this was their work, leveraging their network.
What we discovered is that what mattered was not really that they picked an experienced technical manager, what matter is that the technical manager had supervised in the past a project with a similar technical challenge than the one placed by the new building. The implication of these findings in terms of, let’s say, staffing project was that they had to go back and codify the characteristics, the unique strange characteristics of different buildings and who did them.
Every time a new project was coming up, they knew who were the best qualified technical mentor to lead it. If they were too busy, simply they would have been shadowing the selected technical manager. You may be asking, “Okay, great, they were distributing work, but what about money?” What we found is that selecting a manager with a high level, let’s say, 75 percentile of this related technical expertise compared to a low-level, 25th percentile changed by 13% project margin variation, and you’ll get nice money because these are costly projects. You see that even leadership decision can be–
[00:24:12] Josh: Hey, we’re going to take a real quick break to hear from our sponsors. Stay tuned for more Conquering Chaos.
[00:24:18] Rob: Hey, listeners, it’s Rob. I’m one of the producers on Conquering Chaos. I’m right here with you for every episode, working behind the scenes to make sure everything is just right for your listening experience. Whether you’re a new listener binging content to help you conquer the everyday chaos or a dedicated fan tuning in for each new episode, there’s one thing to always keep in mind, information is useless unless you use it. Obvious, right? It’s so easy to learn, forget, and then miss out on the opportunity to make real improvements to day-to-day activities.
The folks at Parsable have an opportunity for you to learn, experience, and make real improvements to those same day-to-day activities. Get rid of paper on the factory floor. It’s the quickest and easiest way to make a measurable impact on safety, quality and production. Think about it, paper-based checklists, forms, and SOPs isolate workers from getting the information they need when they need it, which leads to a number of inherent inefficiencies that you probably accept as a part of your own everyday chaos.
As a result, you can’t respond quickly to problems. You struggle to standardize the completion of critical tasks, and you miss out on new continuous improvement opportunities. Parsable is proven to help across a number of different functions, including autonomous maintenance, line changeovers, in-process quality checks, and more, which has helped industry-leading manufacturers reduce unplanned downtime, increase OEE, improve throughput, and more.
See for yourself how easy it is to bring a connected digital experience to your frontline workers by using Parsable risk-free for 30 days. Check the show notes for the link. All right, back to the show.
[00:26:25] Josh: That’s an interesting example where it’s a little bit more than just having an experienced individual, an experienced technical manager, it’s taking that further, it’s an experienced technical manager who worked on something very similar, so they’ve built up that experience. It sounds like it now became a factor to look at when considering projects and who the leadership of the particular project should be is who worked on something similar in the past.
[00:26:55] Fabrizio: Right. If you don’t know what, you don’t know what to qualify because sometime the problem for organization is they take shortcut, say, “Hey, this is a difficult project, let’s pick an experienced guy.” That doesn’t explain success. That’s why I said our homo sapiens brains takes too easy shortcuts many times. When it comes people decision, especially leadership decision, they have important consequences on performance.
[00:27:27] Josh: Absolutely. I would love to hear another example. I will say with what you described, it sounds very familiar to me to situations that we encounter here at Parsable where we talk with operations, different leaders at different factory sites who have variation in things like line changeovers, for example, or even starting up a line.
To your earlier point, there’s a lot of variables there, but there’s a consistent situation that we see where there’s a reliance on a specific individual or people with years of experience with either that particular line or machine or similar type of machinery. I’m wondering if there is impacts that people analytics can have on the way in which companies can start to share knowledge because it sounds like you could start by identifying who that ideal person is. Do you see an avenue for how people analytics can really help others understand what knowledge needs to be shared and with whom?
[00:28:42] Fabrizio: This is actually a pretty interesting question that you place. I would love to do a project on this, but I’ve been thinking about this quite a number of time. Josh, the fundamental keyword that you pull out here is knowledge sharing and what type of knowledge is being shared. Traditionally, it was impossible for organization to know this scientifically because basically, they didn’t have data about knowledge sharing, but now situation is different.
Think, for example, Kone Elevators, the Finnish multinational, I think they have more than one million elevators installed globally. Actually, the maintenance is really the moneymaker of the business more than the installation. A few years ago, they created a global system where, let’s say, a technician in Chennai, India could find a problem with an elevator system and figure out a smart solution, type it into the system, this gets automatically translated, and then another elevator maybe in Memphis, can retrieve this by means of artificial intelligence algorithm and then reuse the information.
Now, what is interesting here beyond the simple fact that I have a knowledge sharing tool that works is that the manager of the system can observe that, let’s call it Ram in Chennai, Raman, is a worker that actually shares a lot. Maybe Josh is another worker who shares a lot, but they can also find that yes, Raman shares a lot, but what Josh shares is used a lot by others.
I can understand, I can get information for the first time in history about who’s helping and who’s open to getting help. I can tie this to compensation systems because, Josh, I know that you are 70% among the top in the maintenance jobs so you would get 70% of the bonus, but you are 100% in terms of sharing solutions. You get 90% of the bonus.
This type of analytics is very interesting because it helps fix a problem that organization had forever in collaboration that managers brag about collaboration. You need to collaborate. You need to collaborate but that collaboration is costly. If I can observe collaboration and connect it to incentives, that connection because we know without things incentives, it’s difficult to start to drive people. If all your incentives are on productivity and not collaboration, guess what they will do.
[00:31:52] Josh: Productivity focus. Everything’s about doing the work, nothing about collaboration.
[00:31:58] Fabrizio: In this sense, another interesting example comes from Price Waterhouse Coopers, they were an early mover with robotic process automation and they created in their operational excellence center in Florida. After the first rollout of RPA, they found that the tool was not applied as much as they would liked. They created what they call a citizen-led approach, according to which workers can actually apply and enroll to the RPA program, they get training and they can use robots, but they can also modify them.
Then you can upload your modified robots into PWC platform, previous approval, of course, from the central unit, because they’re there to be sure what they upload, because you are doing audits, and then other people, other workers can get your robot and modify it themselves.
In the end of the day, they link this to the performance review that employee get hearing. Not only how well you’re doing, but also how much you are improving robots and how much people are using your improved robots. This idea of using digital observation of people to create incentive for collaboration and to create basically innovation platform that work bottom-up is fundamental and allows us to realize one holy grill of lean, the idea of bottom-up, scalable improvement.
[00:33:29] Josh: What I love about that is the concepts that you’re bringing up, because the concepts themselves aren’t new, not even with people analytics, it’s that idea of observing, understanding, making a change and then making efforts to promote a positive reception to that change. In this case, using the data about not just what information is being shared, which we could probably take a little further and say with the right systems in place, you can capture data on the impact of that information that was shared, but also who is sharing that information and who is receptive to the information shared by whom.
There’s, again, a lot of different variables to consider in this scenario of knowledge share but that key concept that you brought up of observing, making decisions, observing the impact of those decisions, just a constant cycle there and connecting to a reward, connecting to how this had the positives that in enable people to adopt it or that get people on board to adopt and continue to reproduce the behavior that you’re looking for is something that can’t be overlooked.
In fact, most of the digital projects and initiatives I’ve discussed with people here, both on this podcast as well as just in conversations in industry, a lot of the failure year comes from the change management side, getting people bought in and becoming active participants and not just active participants, but people that want to participate who see the value in some way of changing what they were doing before to what that more desired behavior is.
That actually leads to the next section that I wanted to pick your brain about because we covered with people analytics, we talked about what it is and we’ve talked about why it’s important, but let’s talk about the how. It’s not enough to know that people analytics is a tool that can help leaders in ways that they may not traditionally have expected. We need to make sure that we’re empowering our listeners or our students for today with the know-how that’s needed to get started. Fabrizio, how do you get started with people analytics used in this way?
[00:36:01] Fabrizio: How do you get started? There are two inputs of the problem of the process of setting up people analytics solutions. I would say that always the starting point is what is the people related problem that the organization want to address? Never start with algorithms, start with business problems, in this case, people-related issues. That is always something that you must keep in mind.
Imagine I have a tendering organization for a telecom company, a project that we closed last year and they’re doing 30,000 offers to business-to-business clients. It’s clear that I would like to create highly effective teams on offers that generate successful bids. That it’s very simple. Everybody can understand it. Then you go back and say, okay, maybe not all teams work equally well. Certainly, there are aspects of team chemistry that we cannot capture with analytics, but there are aspect that we can.
The question therefore goes to what is the data mesh that we can create within the organization about this? This apparently is obvious, but in my experience is actually one of the real pain points because if you read Harvard Business Review, it seems that all organization are like Star Trek, spaceship, clean, shiny, full of data and everything is perfect but then when you go in reality, they look more like Star Wars starship and not the big one of the buyer, the small one of the rebels with a lot of stuff that is not well connected, things that better not to look there. Just this step is very important.
The step is okay, what is that I have to address the question that I have? Do I have enough information? That is the first experiment that if you want to do if you need to get into the people analytics is okay, let’s try to put together a data dimension, how the costly is it and how many holes it has. Then we’ll show it to a data scientist. Let’s see whether we can get something from that step, right?
An important thing that I would like to say to all the listeners here is that I don’t obsessed by big data because sometimes small data is pretty insightful. If you want to do analytics in a project-based organization, like an R&D facility, a high tech project special unit, you won’t have more than a few hundred cumulated projects across a few years. That’s small data, but it’s not so small that you can do inferences.
The second important message here is don’t be too critical with your data. In my experience, managers are very self-critical. Our data is full of errors. There is this and there is that. Well, if you have enough data and you know what there are, you can model them into algorithms and don’t be too negative about the quality of your data. A lot of stuff can be done there.
At this point, imagine that you have the data match. You say, Hey, well, what do I do? The second big question that I get from managers is how the hell can I prove the value of my analytics idea to upper management? How did I get cleared for doing this? Quite simply, oftentimes simply you can’t because you should be making so many assumptions about the effects of these things that are very hard they authorize a pilot. Between having a data mess and doing a pilot that is an intermediate stage which you can either call it experiments or you can call it proof for value, the idea is that we don’t do anything for real. We just check at least a couple of things.
One, can I make reliable forecast or predictions based on the data that I have? Imagine that I want to forecast, for example, the probability that an employee will leave the organization if I assign him to project A rather than project B. You can actually do this and test it again, train on a data set and test on other data of the same company and see whether you can predict it. First of all, is this data useful to support our question?
Then there is a second problem which is, I have the algorithm but the algorithm is something is a data structure. How this algorithm would sit on the desk of people in the organization, how would it interact with their day to day job?
At this stage, you need to think about how the interface of this algorithm with real users would look like and check this with users but also with IT because if you ask IT general question about an algorithm, it’s very likely that they come out with a lot of problems but if you show no, can you code this interface that is based on this, if then rule whatever? Well, if they say no, they better have a good answer. Turning this into the positive before launching the pilot is important to check with these, let’s say, simulation of screens or interfaces, how people react to the new innovation and also create excitement.
Because then I saw when I saw to HR of a multinational technology and let’s say consulting this algorithm that was actually predicting with 89% accuracy whether a worker is going to leave the company the following year, they were really looking attentively because, hey, how do you do that?
When you’re done with this proof of concept, then you’re ready for the pilot which is in the language of entrepreneurship, a minimum viable product and what you check there is whether the thing can really work in a real office or in a real plant or in a real supply chain. When these work, then you have the final step, scale, right? Can I roll it across all my units, all my plants, all my offices, whatever is the target unit. If you say these are the steps, data meshes, experiments, pilots, scaling or if you want, data meshes, proof of value, minimal via viable products and global solutions schedule.
[00:43:16] Josh: From what you said, there’s not a need to start big. In fact, from what you’re saying, the more specific you can get with the problem that you’re trying to solve and start there, that’s what sets you up for greater success in the long run. Really it’s that idea of formulating the hypothesis, understanding what it is you need to actually test this hypothesis to either validate or invalidate it, and then understand why, whichever way it goes and then take those learnings and then roll it into whatever the next project is.
I love that idea of you got to get specific. It doesn’t have to be big. Start small, start with a specific problem. Let that define the data points that you then need so that you can understand where you can get those data points to then bring that in and successfully conduct an experiment or like you said, a proof of value also sometimes called the proof of concept just to prove that this is something that could work for us. Then from there, making sure you get, for example, IT and senior leadership on board.
I can’t tell you how many projects I’ve discussed with people where that question of ROI comes up. I think it’s valid. People want to make sure they’re making wise financial investments and in certain situations, it’s difficult to estimate the value or to prove that there will be a value because you have to test it first and you have to be okay with failing from time to time. When I say failing, I mean the projects not turning into anything greater than it was. You can look at that as a failure but really everyone should look at that as a success because you have to be testing to see what’s going to work, why, so that you can future proof your, your organization.
I think that’s very solid advice in that’s not just people analytics with what you’re describing, that’s solid advice to take for really any initiative that you’re planning. That is the blueprint. Listeners, you just got a masterclass on implementation in change management right there. Certainly there’s more to it especially with the buy-in but those concepts, those are the key milestones to really focus on. Now, last question I have for you, Fabrizio, is really about how our listeners can continue the conversation or how you can help if they reach out.
[00:45:51] Fabrizio: Thank you for asking also this question. I normally interact with a lot of practitioners and the more I had in my career, the more I do that not only for developing business or whatever, it’s just out of those intellectual curiosity. People who will want to reach me, they’re very welcome and I will be happy to provide assessment on let’s say– Well, my area really of expertise is people analytics but I’m also quite advanced, I would say, in Industry 4.0 and this type of initiatives.
Also, people are very interested in sharing success or failure stories. If someone listen to these and say, hey, you know what? I think that you’re right but people analytics can be extremely tricky because and because or I find a type of implementation which is different from what I provide here as examples. Super welcome. That would be fantastic. Of course, explore possible collaborations for helping you thinking through this, coaching, thinking about working with your team about how define a roadmap for people analytics or similar type of problems. These are all things that I do. Of course, collaborative research projects. I can involve my teams and it would be another type of engagement, pretty open.
[00:47:27] Josh: What’s the best way to reach out to you?
[00:47:29] Fabrizio: Well to reach me as just LinkedIn is the universal business interface. I will pass my email to Josh later, if someone wants to link through him or through the information that he will put on the web. I would say that LinkedIn, that’s the simplest way to reach me quite frankly.
[00:47:54] Josh: Great. We’ll be sure to do that. I’m definitely going to keep in contact with you. I really encourage listeners, reach out, even if it’s like Fabrizio said, sharing successes, sharing opinions and even if you disagree, that’s okay too. Let’s hear about it because the more perspectives that we can get, the more we can work together to build a shared understanding that helps bring everyone to similar successes in the future. With that, Fabrizio, thank you so much for joining us today.
[00:48:24] Fabrizio: My pleasure, Josh. Thank you for the invitation. Thank you for the very entertaining conversation that we had and all the best for your next interviews.
[00:48:37] Josh: Thank you. I appreciate that.
[00:48:41] Walt: Hey all, it’s Walt, here. I’m another producer for Conquering Chaos. Before you go, if you’re not ready to try Parsable to help you get rid of paper, why not watch a quick video instead, check the show notes for a link to a demonstration Josh put together to show frontline workers what it’s like to use a dynamic digital experience to get work done. In it, Josh shows you how using a modern day app enables you to connect to people, information, systems and machines, just like the apps you use in your personal lives. Take a look and let us know what you think.
[00:49:16] Josh: That’s the show. Thank you so, so much for joining us today. Conquering Chaos is brought to you by Parsable. If you’re a fan of these conversations, subscribe to the show and leave us a rating on apple podcast, just tap the number of stars you think the show deserves. As always, feel free to share what’s top of mind for you and who you think we should talk to next. Until then, talk soon, take care, stay safe. Bye-bye.
Listen to find out why manufacturing needs people analytics